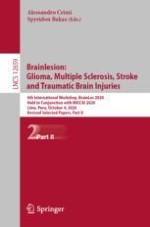
2021 | OriginalPaper | Chapter
Lightweight U-Nets for Brain Tumor Segmentation
Authors : Tomasz Tarasiewicz, Michal Kawulok, Jakub Nalepa
Published in: Brainlesion: Glioma, Multiple Sclerosis, Stroke and Traumatic Brain Injuries
Publisher: Springer International Publishing
Activate our intelligent search to find suitable subject content or patents.
Select sections of text to find matching patents with Artificial Intelligence. powered by
Select sections of text to find additional relevant content using AI-assisted search. powered by