Abstract
Accurate prediction of shear stress distribution along the boundary in an open channel is the key to solving numerous critical engineering problems such as flood control, sediment transport, riverbank protection, and others. Similarly, the estimation of flow discharge in flood conditions is also challenging for engineers and scientists. The flow structure in compound channels becomes complicated due to the transfer of momentum between the deep main channel and the adjoining floodplains, which affects the distribution of shear force and flow rate across the width. Percentage sharing of shear force at floodplain (%Sfp) is dependent on the non-dimensional parameters like width ratio of the channel \((\alpha )\), relative depth \((\beta )\), sinuosity \((s)\), longitudinal channel bed slope \((S_{{\text{o}}} ),\) meander belt width ratio \((\omega )\), and differential roughness \((\gamma )\). In this paper, various artificial intelligence approaches such as multivariate adaptive regression spline (MARS), group method of data handling Neural Network (GMDH-NN), and gene-expression programming (GEP) are adopted to construct model equations for determining %Sfp for meandering compound channels with relative roughness. The influence of each parameter used in the model for predicting the %Sfp is also analyzed through sensitivity analysis. Statistical indices are employed to assess the performance of these models. Validation of the developed %Sfp model is performed for the experimental observations by conventional analytical models; to verify their effectiveness. Results indicate that the proposed GMDH-NN model predicted the %Sfp satisfactorily with the coefficient of determination (R2) of 0.98 and 0.97 and mean absolute percentage error (MAPE) of 0.05% and 0.04% for training and testing dataset, respectively as compared to GEP and MARS. The developed model is also validated with various sinuous channels having sinuosity 1.343, 1.91 and 2.06.






Similar content being viewed by others
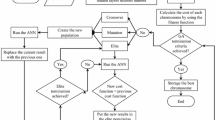
Data Availability
All data, models, and code generated or used during the study appear in the submitted article. In detail data can be found from the relevant literatures.
References
Ackers P (1993) Stage-discharge functions for two-stage channels: the impact of new research. Water Environ J 7(1):52–59
Adamowski J, Chan HF, Prasher SO, Sharda VN (2012) Comparison of multivariate adaptive regression splines with coupled wavelet transform artificial neural networks for runoff forecasting in Himalayan micro-watersheds with limited data. J Hydroinf 14(3):731–744
Akay H (2021a) Flood hazards susceptibility mapping using statistical, fuzzy logic, and MCDM methods. Soft Comput 1–22
Akay H (2021b) Spatial modeling of snow avalanche susceptibility using hybrid and ensemble machine learning techniques. CATENA 206:105524
Amanifard N, Nariman-Zadeh N, Farahani MH, Khalkhali A (2008) Modeling of multiple short-length-scale stall cells in an axial compressor using evolved GMDH neural networks. Energy Convers Manag 49(10):2588–2594
Arcement GJ, Schneider VR (1989) Guide for selecting Manning's roughness coefficients for natural channels and flood plains. US Government Printing Office Washington, DC
Berlamont JE, Trouw K, Luyckx G (2003) Shear stress distribution in partially filled pipes. J Hydraul Eng 129(9):697–705
Bhattacharya AK (1995) Mathematical model of flow in a meandering channel. IIT Kharagpur
Bonakdari H, Baghalian S, Nazari F, Fazli M (2011) Numerical analysis and prediction of the velocity field in curved open channel using artificial neural network and genetic algorithm. Eng Appl Comput Fluid Mech 5(3):384–396
Christensen HB, Fredsoe J (1998) Bed shear stress distribution in straight channels with arbitrary cross section
Cobaner M, Seckin G, Seckin N, Yurtal R (2010) Boundary shear stress analysis in smooth rectangular channels and ducts using neural networks. Water Environ J 24(2):133–139
Craven P, Wahba G (1978) Smoothing noisy data with spline functions. Numer Math 31(4):377–403
Das AK (1984) A study of river flood plain interaction and boundary shear stress distribution in a meander channel with one sided flood plain. Ph. D, Indian Institute of Technology Kharagpur, Kharagpur, India
Friedman JH (1991) Multivariate adaptive regression splines. Ann Stat 1–67
Ferreira C (2001) “Algorithm for solving gene expression programming: a new adaptive problem.” Complex Systems 13(2):87-129
Ferreira C (2002) “Gene expression programming in problem solving.” In: Soft computing and industry, Springer, 635-653
Friedman JH, Roosen CB (1995) “An introduction to multivariate adaptive regression splines.” Sage Publications Sage CA: Thousand Oaks, CA
Gholamnia K, Gudiyangada Nachappa T, Ghorbanzadeh O, Blaschke T (2020) Comparisons of diverse machine learning approaches for wildfire susceptibility mapping. Symmetry 12(4):604
Ghosh S, Jena SB (1971) Boundary shear distribution in open channel compound. Proc Inst Civ Eng 49(4):417–430
James CS, Wark JB (1992) Conveyance estimation for meandering channels. SR329, HR Wallingford
Kar SK (1977) A study of distribution of boundary shear in meander channel with and without floodplain and river floodplain interaction. Ph. D Indian Institute of Technology Kharagpur, Kharagpur, India
Khatua KK (2007) Interaction of flow and estimation of discharge in two stage meandering compound channels. Ph.D, National Institute of Technology Rourkela, Odisha, India
Khatua KK, Patra KC (2007) Boundary shear stress distribution in compound open channel flow. ISH J Hydraul Eng 13(3):39–54
Khatua KK, Patra KC, Mohanty PK (2011a) Stage-discharge prediction for straight and smooth compound channels with wide floodplains. J Hydraul Eng 138(1):93–99
Khatua KK, Patra KC, Nayak P (2011b) Meandering effect for evaluation of roughness coefficients in open channel flow. WIT Trans Ecol Environ 146:213–224
Khatua KK, Patra KC, Nayak P, Sahoo N (2012) “Stage-discharge prediction for meandering channels.” International Journal of Computational Methods and Experimental Measurements 1(1):80-92
Knight DW (1981) Boundary shear in smooth and rough channels. J Hydraul Div 107(7):839–851
Knight DW, Demetriou JD (1983) Flood plain and main channel flow interaction. J Hydraul Eng 109(8):1073–1092
Knight DW, Hamed ME (1984) Boundary shear in symmetrical compound channels. J Hydraul Eng 110(10):1412–1430
Knight DW, Sterling M (2000) Boundary shear in circular pipes running partially full. J Hydraul Eng 126(4):263–275
Koza JR (1992) “Genetic programming: On the programming of computers by means of natural selection. MA.” MIT Press, Cambridge
Leighly JB (1932) Toward a theory of the morphologic significance of turbulence in the flow of water in streams. University of California Press
Leutheusser HJ (1963) Turbulent flow in rectangular ducts. J Hydraul Div 89(3):1–19
Mallick M, Mohanta A, Kumar A (2020a) Multivariate adaptive regression spline approach to the assessment of surface mean pressure coefficient on surfaces of C-shaped building. Sci Iran 27(6):2967–2984
Mallick M, Mohanta A, Kumar A, Charan Patra K (2020b) Prediction of wind-induced mean pressure coefficients using GMDH neural network. J Aerosp Eng 33(1):04019104
Mallick M, Mohanta A, Kumar A, Patra KC (2020c) Gene-expression programming for the assessment of surface mean pressure coefficient on building surfaces. Build Simul 13(2):401–418
Mehdizadeh S, Behmanesh J, Khalili K (2017a) Application of gene expression programming to predict daily dew point temperature. Appl Therm Eng 112:1097–1107
Mehdizadeh S, Behmanesh J, Khalili K (2017b) Using MARS, SVM, GEP and empirical equations for estimation of monthly mean reference evapotranspiration. Comput Electron Agric 139:103–114
Milukow HA, Binns AD, Adamowski J, Bonakdari H, Gharabaghi B (2018) Estimation of the darcy-weisbach friction factor for ungauged streams using gene expression programming and extreme learning machines. J Hydrol 568:311–321
Mohanta A, Patra KC (2019) MARS for prediction of shear force and discharge in two-stage meandering channel. J Irrig Drain Eng 145(8):04019016
Mohanta A, Patra KC (2021) Gene-expression programming for calculating discharge in meandering compound channels. Sustain Water Res Manag 7(3):33
Mohanta A, Patra KC, Sahoo B (2018) Anticipate Manning’s coefficient in meandering compound channels. Hydrology 5(3):47
Mohanta A, Patra KC, Pradhan A (2020) Enhanced channel division method for estimation of discharge in meandering compound channel. Water Resour Manag 34(3):1047–1073
Mohanty PK (2013) Flow analysis of compound channels with wide flood plains prabir. PhD., National Institute of Technology Rourkela, Odisha, India
Mosavi A, Hosseini FS, Choubin B, Goodarzi M, Dineva AA, Sardooi ER (2021) Ensemble boosting and bagging based machine learning models for groundwater potential prediction. Water Resour Manag 35(1):23–37
Myers WRC (1987) Velocity and discharge in compound channels. J Hydraul Eng 113(6):753–766
Nachappa TG, Ghorbanzadeh O, Gholamnia K, Blaschke T (2020) Multi-hazard exposure mapping using machine learning for the State of Salzburg, Austria. Remote Sens 12(17):2757
Najafzadeh M, Azamathulla HM (2013) Neuro-fuzzy GMDH to predict the scour pile groups due to waves. J Comput Civ Eng 29(5):04014068
Najafzadeh M, Lim SY (2015) Application of improved neuro-fuzzy GMDH to predict scour depth at sluice gates. Earth Sci Inf 8(1):187–196
Najafzadeh M, Rezaie-Balf M, Tafarojnoruz A (2018) Prediction of riprap stone size under overtopping flow using data-driven models. Int J River Basin Manag 16(4):1–8
Newcombe RG (1998) Two-sided confidence intervals for the single proportion: comparison of seven methods. Stat Med 17(8):857–872
Noutsopoulos G, Hadjipanos P (1983). Discharge computations in compound channels. Proc. 20th IAHR Congress, 173–180
Patra KC, Kar SK (2000) Flow interaction of meandering river with floodplains. J Hydraul Eng 126(8):593–604
Patra KC, Kar SK, Bhattacharya AK (2004) Flow and velocity distribution in meandering compound channels. J Hydraul Eng 130(5):398–411
Patra KC (1999) Flow interaction of meandering river with flood plains. Doctor of Philosophy, Indian Institute of Technology Kharagpur, Kharagpur, India
Pradhan A, Khatua KK (2017) Gene expression programming to predict Manning’s n in meandering flows. Can J Civ Eng 45(4):304–313
Pradhan A (2019) Stage-discharge modelling of meandering compound channels with differential roughness. PhD., National Institute of Technology Rourkela, Odisha, India
Prinos P, Townsend RD (1984) Comparison of methods for predicting discharge in compound open channels. Adv Water Resour 7(4):180–187
Sattar AMA (2013) Gene expression models for the prediction of longitudinal dispersion coefficients in transitional and turbulent pipe flow. J Pipeline Syst Eng Pract 5(1):04013011
Stephenson D, Kolovopoulos P (1990) Effects of momentum transfer in compound channels. J Hydraul Eng 116(12):1512–1522
Toebes GH, Sooky AA (1967) Hydraulics of meandering rivers with flood plains. J Waterways Harbors Division 93(2):213–236
Willetts BB, Hardwick RI (1993) Stage dependency for overbank flow in meandering channels. Proc Instit Civil Eng-Water Maritime and Energy 101(1):45–54
Wormleaton PR, Hadjipanos P (1985) Flow distribution in compound channels. J Hydraul Eng 111(2):357–361
Wormleaton PR, Hadjipanos P, Allen J (1980) Discussion of iInteraction between main channel and flood-plain flows. J Hydraul Div 106(5):942–942
Wormleaton PR, Allen J, Hadjipanos P (1982) Discharge assessment in compound channel flow. J Hydraul Div 108(9):975–994
Yang S-Q, Lim S-Y (2005) Boundary shear stress distributions in trapezoidal channels. J Hydraul Res 43(1):98–102
Yen C-L, Overton DE (1973) Shape effects on resistance in flood-plain channels. J Hydraul Div 99(1):219–238
Yu G, Tan S-K (2007) Estimation of boundary shear stress distribution in open channels using flownet. J Hydraul Res 45(4):486–496
Zahiri A, Eghbali P (2012) Gene expression programming for prediction of flow discharge in compound channels. J Civil Eng Urbanism 2(4):164–169
Acknowledgements
The authors acknowledge the support received from the Department of Civil Engineering, National Institute of Technology Rourkela, India.
Funding
This research received no external funding.
Author information
Authors and Affiliations
Contributions
AM and AP have done the experimental investigation and collected the data. AM and AP has done the analysis and validation of the experimental results. AM, AP and MM developed the model and analyzed the results under the supervision of KCP. KCP reviewed the work and provided feedback on this paper.
Corresponding author
Ethics declarations
Ethical Approval
Not applicable.
Consent to Participate
Not applicable.
Consent to Publish
Not applicable.
Conflict of Interest
The authors declare no conflict of interest.
Additional information
Publisher's Note
Springer Nature remains neutral with regard to jurisdictional claims in published maps and institutional affiliations.
Rights and permissions
About this article
Cite this article
Mohanta, A., Pradhan, A., Mallick, M. et al. Assessment of Shear Stress Distribution in Meandering Compound Channels with Differential Roughness Through Various Artificial Intelligence Approach. Water Resour Manage 35, 4535–4559 (2021). https://doi.org/10.1007/s11269-021-02966-5
Received:
Accepted:
Published:
Issue Date:
DOI: https://doi.org/10.1007/s11269-021-02966-5