Abstract
Discernment of seismic soil liquefaction is a complex and non-linear procedure that is affected by diversified factors of uncertainties and complexity. The Bayesian belief network (BBN) is an effective tool to present a suitable framework to handle insights into such uncertainties and cause–effect relationships. The intention of this study is to use a hybrid approach methodology for the development of BBN model based on cone penetration test (CPT) case history records to evaluate seismic soil liquefaction potential. In this hybrid approach, naive model is developed initially only by an interpretive structural modeling (ISM) technique using domain knowledge (DK). Subsequently, some useful information about the naive model are embedded as DK in the K2 algorithm to develop a BBN-K2 and DK model. The results of the BBN models are compared and validated with the available artificial neural network (ANN) and C4.5 decision tree (DT) models and found that the BBN model developed by hybrid approach showed compatible and promising results for liquefaction potential assessment. The BBN model developed by hybrid approach provides a viable tool for geotechnical engineers to assess sites conditions susceptible to seismic soil liquefaction. This study also presents sensitivity analysis of the BBN model based on hybrid approach and the most probable explanation of liquefied sites, owing to know the most likely scenario of the liquefaction phenomenon.
摘要
地震液化评估是一个复杂的非线性过程,受多种因素的不确定性和复杂性的影响。贝叶斯置信 网络(BBN)是一个可靠有效的工具,可以提供一个合适的框架来处理这些不确定性和因果关系。本研 究采用一种混合方法来建立基于静力触探试验(CPT)案例记录数据的贝叶斯置信网络(BBN)模型,以评 估土壤的地震液化势。在这种混合方法中,先通过结合领域知识(DK)的解释结构建模(ISM)技术建立 朴素模型,再在K2 算法中嵌入朴素模型的相关信息建立BBN-K2 和DK 模型。将BBN 模型的结果 与现有的人工神经网络(ANN)和 C4.5 决策树(DT)模型进行了比较和验证,发现用混合方法建立的 BBN 模型在液化势评估中具有良好的适应性和应用前景。用混合方法建立的BBN 模型为岩土工程师评估 易受地震液化影响的场地环境提供了可行的工具。最后对基于混合方法的BBN 模型进行了灵敏度分 析,并对液化场地进行了最可能的解释,以了解液化现象的最可能情况。
Similar content being viewed by others
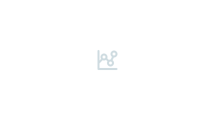
References
GOH ANTHONY T C. Seismic liquefaction potential assessed by neural networks [J]. Journal of Geotechnical Engineering, 1994, 120(9): 1467–1480. DOI: 10.1016/0148-9062(95)99150-V.
GOH ANTHONY T C. Neural-network modeling of CPT seismic liquefaction data [J]. Journal of Geotechnical Engineering, 1996, 122(1): 70–73. DOI: 10.1061/(ASCE)0733-9410(1996)122:1(70).
JUANG C H, CHEN Jin-xia, TIEN Yong-ming. Appraising cone penetration test based liquefaction resistance evaluation methods: artificial neural network approach [J]. Canadian Geotechnical Journal, 1999, 36(3): 443–454. DOI: 10.1139/t99-011.
GOH ANTHONY T C, GOH S H. Support vector machines: their use in Geotechnical engineering as illustrated using seismic liquefaction data [J]. Computer and Geotechnics, 2007, 34(5): 410–421. DOI: 10.1016/j.compgeo.2007.06.001.
PAL M. Support vector machines-based modeling of seismic liquefaction potential [J]. International Journal for Numerical and Analytical Methods in Geomechanics, 2006, 30(10): 983–996. DOI: 10.1002/nag.509.
SAMUI P. Least square support vector machine and relevance vector machine for evaluating seismic liquefaction potential using SPT [J]. Natural Hazards, 2011, 59(2), 811–822. DOI: 10.1007/s11069-011-9797-5.
SAMUI P. Seismic liquefaction potential assessment by using relevance vector machine [J]. Earthquake Engineering and Engineering Vibration}, 6(4): 331-336. DOI: 10.1007/s11803-007-0766-7.
ZHOU Jian, LI En-ming, WANG Ming-zheng, CHEN Xin. Feasibility of stochastic gradient boosting approach for evaluating seismic liquefaction potential based on SPT and CPT case histories [J]. Journal of Performance of Constructed Facilities, 2019, 33(3): 1–10. DOI: 10.1061/(ASCE)CF.1943-5509.0001292.
MUDULI P M, DAS S K. CPT-based seismic liquefaction potential evaluation using multi-gene genetic programming approach [J]. Indian Geotechnical Journal, 2014, 44(1): 86–93. DOI: 10.1007/s40098-013-0048-4.
GANDOMI A H, ALAVI A H. Hybridizing genetic programming with orthogonal least squares for modeling of soil liquefaction [J]. International Journal of Earthquake Engineering and Hazard Mitigation, 2013, 1(1): 2–8.
LIANG Wan-jie, ZHUANG Da-fang, JIANG Dong, PAN Jian-jun, REN Hong-yan. Assessment of debris flow hazards using a Bayesian network [J]. Geomorphology, 2012, 171: 94–100. DOI: 10.1016/j.geomorph.2012.05.008.
PEARL J. Probabilistic reasoning in intelligent systems [M]. San Mateo, California: Morgan Kaufmann Publishers, 1988. DOI: 10.1016/C2009-0-27609-4.
COCKBURN G, TESFAMARIAM S. Earthquake disaster risk index for Canadian cities using Bayesian belief networks [J]. Georisk: Assessment and Management of Risk for Engineered Systems and Geohazards, 2012, 6(2): 128–140. DOI: 10.1080/17499518.2011.650147.
BENSI M T, DER K A, STRAUB D. A Bayesian network framework for post-earthquake infrastructure system performance assessment [C]// Technical Council on Lifeline Earthquake Engineering Conference (TCLEE) 2009: Lifeline Earthquake Engineering in a Multihazard Environment. Oakland, CA, 2009: 1097–1107.
BAYRAKTARLI Y Y, BAKER J W, FABER M H. Uncertainty treatment in earthquake modelling using Bayesian probabilistic networks [J]. Georisk: Assessment and Management of Risk for Engineered Systems and Geohazards, 2011, 5(1): 44–58. DOI: 10.1080/ 17499511003679931.
FAIZIAN M, SCHALCHER H R, FABER M H. Consequence assessment in earthquake risk management using damage indicators [C]// First International Forum on Engineering Decision Making (IFED). Stoos, Switzerland, 2004.
TESFAMARIAM S, LIU Z. Earthquake induced damage classification for reinforced concrete buildings [J]. Structural Safety, 2010, 32(2): 154–164. DOI: 10.1016/j.strusafe.2009.10.002.
BAYRAKTARLI Y Y, FABER M H. Bayesian probabilistic network approach for managing earthquake risks of cities [J]. Georisk: Assessment and Management of Risk for Engineered Systems and Geohazards, 2011, 5(1): 2–24. DOI: 10.1080/17499511003679907.
LI Lian-fa, WANG Jin-feng, LEUNG H, JIANG Chang-sheng. Assessment of catastrophic risk using Bayesian network constructed from domain knowledge and spatial data [J]. Risk Analysis, 2010, 30(7): 1157–1175. DOI: 10.1111/j.1539-6924.2010.01429.x.
SCHUBERT M, FABER M H. Common cause effects in portfolio loss estimation [J]. Structure and Infrastructure Engineering, 2011, 8(5): 497–506. DOI: 10.1080/15732479.2010.539068.
NISHIJIMA K, MAES M A, GOYET J, FABER M H. Constrained optimization of component reliabilities in complex systems [J]. Structural Safety, 2009, 31(2), 168–178. DOI: 10.1016/j.strusafe.2008.06.016.
STRAUB D, KIUREGHIAN ARMEN D. Bayesian network enhanced with structural reliability methods: Methodology [J]. Journal of Engineering Mechanics, 2010, 136(10): 1248–1258. DOI: 10.1061/(ASCE)EM.1943-7889.0000173.
STRAUB D, KIUREGHIAN ARMEN D. Combining Bayesian networks with structural reliability methods: application [J]. Journal of Engineering Mechanics, 2010, 136(10): 1259–1270. DOI: 10.1061/(ASCE)EM.1943-7889.0000170.
WEBER P, MEDINA- OLIVA G, SIMON C, IUNG B. Overview on Bayesian networks applications for dependability, risk analysis and maintenance areas [J]. Engineering Applications of Artificial Intelligence, 2010, 25(4): 671–682. DOI: 10.1016/j.engappai.2010.06.002.
JUANG C H, JIANG Tao, ANDRUS R D. Assessing probability-based methods for liquefaction potential evaluation [J]. Journal of Geotechnical and Geoenvironmental Engineering, 2002, 128: 580–589. DOI: 10.1061/(ASCE)1090-0241(2002)128:7(580).
BAYRAKTARLI Y Y. Application of Bayesian probabilistic networks for liquefaction of soil [C]// 6th International PhD Symposium in Civil Engineering. Zurich, 2006.
HUANG H W, ZHANG J, ZHANG L M. Bayesian network for characterizing model uncertainty of liquefaction potential evaluation models [J]. KSCE Journal of Civil Engineering, 2012, 16(5): 714–722. DOI: 10.1007/s12205-012-1367-1.
HU Ji-lei, TANG Xiao-wei, QIU Jiang-nan. A Bayesian network approach for predicting seismic liquefaction based on interpretive structural modeling [J]. Georisk: Assessment and Management of Risk for Engineered Systems and Geohazards, 2015, 9(3): 200–217. DOI: 10.1080/17499518.2015.1076570.
WARFIELD J W. Developing inter connected matrices in structural modeling [J]. IEEE Transactions on Systems, Man, and Cybernetics, 1974, 4(1): 81–87.
AHMAD M, TANG Xiao-wei, QIU Jiang-nan, AHMAD F. Interpretive structural modeling and MICMAC analysis for identifying and benchmarking significant factors of seismic soil liquefaction [J]. Applied Sciences, 2019, 9(2): 233. DOI: 10.3390/app9020233.
PFOHL H C, GALLUS P, THOMAS D. Interpretive structural modeling of supply chain risks [J]. International Journal of Physical Distribution & Logistics Management, 2011, 41(9): 839–859. DOI: 10.1108/09600031111175816.
SAXENA J P, SUSHIL V P. MICMAC analysis for energy conservation [J]. Systems Research, 1990, 7(4): 245–253. DOI: 10.1002/sres.3850070404.
SUSHIL S. Interpreting the interpretive structural model [J]. Global Journal of Flexible Systems Management, 2012, 13: 87–106. DOI: 10.1007/s40171-012-0008-3.
AHMAD M, TANG X W, QIU J N, AHMAD F, GU W. Application of machine learning algorithms for evaluation of seismic soil liquefaction potential [J]. Frontiers of Structural and Civil Engineering. (in press)
COOPER G, HERSKOVITS E. A Bayesian method for the induction of probabilistic networks from data [J]. Machine Learning, 1992, 9: 309–347. DOI: 10.1007/BF00994110.
ARDAKANI A, KOHESTANI V R. Evaluation of liquefaction potential based on CPT results using C4.5 decision tree [J]. Journal of AI and Data Mining, 2015, 3(1): 85–92. DOI:10.5829/idosi.JAIDM.2015.03.01.09.
ZHANG Lian-yang. Predicting seismic liquefaction potential of sands by optimum seeking method [J]. Soil Dynamics and Earthquake Engineering, 1998, 17: 219–226. DOI: 10.1016/S0267-7261(98)00004-9.
HU Ji-lei, TANG Xiao-wei, QIU Jiang-nan. Assessment of seismic liquefaction potential based on Bayesian network constructed from domain knowledge and history data [J]. Soil Dynamics and Earthquake Engineering, 2016, 89: 49–60. DOI: 10.1016/j.soildyn.2016.07.007.
LI Lian-fa, WANG Jin-feng, LEUNG H, JIANG Cheng-sheng. Assessment of catastrophic risk using Bayesian network constructed from domain knowledge and spatial data [J]. Risk Analysis, 2010, 30(7): 1157–1175. DOI: 10.1111/j.1539-6924.2010.01429.x.
WITTEN I H, FRANK E, HALL M A. Data mining: Practical machine learning tools and techniques [M]. Burlington, MA: Elsevier, 2011. DOI: 10.1016/C2009-0-19715-5.
WEI Jiang-tao, HUANG Du-ruo, WANG Gang. Micro-scale descriptors for particle-void distribution and jamming transition in pre- and post-liquefaction of granular soils [J]. Journal of Engineering Mechanics, 2018, 144(8): 04018067. DOI: 10.1061/(ASCE)EM.1943-7889.0001482.
WANG Gang, WEI Jiang-tao. Microstructure evolution of granular soils in cyclic mobility and post liquefaction process [J]. Granular Matter, 2016, 18: 51. DOI: 10.1007/s10035-016-0621-5.
WANG Gang, XIE Yong-ning. Modified bounding surface hypoplasticity model for sands under cyclic loading [J]. Journal of Engineering Mechanics ASCE, 2014, 140(1): 91–101. DOI: 10.1061/(ASCE)EM.1943-7889.0000654.
YE Jian-hong, HUANG Du-ruo, WANG Gang. Nonlinear simulation of offshore breakwater on sloping liquefied seabed [J]. Bulletin of Engineering Geology and the Environment, 2016, 75: 1215–1225. DOI: 10.1007/s10064-016-0906-2.
KAVEH A, HAMZE-ZIABARI S M, BAKHSHPOORI T. Patient rule-induction method for liquefaction potential assessment based on CPT data [J]. Bulletin of Engineering Geology and the Environment, 2018, 77(2): 849–865. DOI: 10.1007/s10064-016-0990-3.
Author information
Authors and Affiliations
Corresponding author
Additional information
Foundation item: Projects(2016YFE0200100, 2018YFC1505300-5.3) supported by the National Key Research & Development Plan of China; Project(51639002) supported by the Key Program of National Natural Science Foundation of China
Rights and permissions
About this article
Cite this article
Mahmood, A., Tang, Xw., Qiu, Jn. et al. A hybrid approach for evaluating CPT-based seismic soil liquefaction potential using Bayesian belief networks. J. Cent. South Univ. 27, 500–516 (2020). https://doi.org/10.1007/s11771-020-4312-3
Received:
Accepted:
Published:
Issue Date:
DOI: https://doi.org/10.1007/s11771-020-4312-3
Key words
- Bayesian belief network
- cone penetration test
- seismic soil liquefaction
- interpretive structural modeling
- structural learning