Abstract
In this study, normal EEG series recorded from healthy volunteers and epileptic EEG series recorded from patients within and without seizure are classified by using Multilayer Neural Network (MLNN) architectures with respect to several time domain entropy measures such as Shannon Entropy (ShanEn), Log Energy Entropy (LogEn), and Sample Entropy (Sampen). In tests, the MLNN is performed with several numbers of neurons for both one hidden layer and two hidden layers. The results show that segments in seizure have significantly lower entropy values than normal EEG series. This result indicates an important increase of EEG regularity in epilepsy patients. The LogEn approach, which has not been experienced in EEG classification yet, provides the most reliable features into the EEG classification with very low absolute error as 0.01. In particular, the MLNN can be proposed to distinguish the seizure activity from the seizure-free epileptic series where the LogEn values are considered as signal features that characterize the degree of EEG complexity. The highest classification accuracy is obtained for one hidden layer architecture.



Similar content being viewed by others
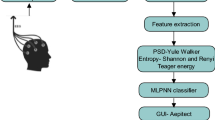
References
Andrzejak, R. G., K. Lehnertz, et al. Indications of nonlinear deterministic and finite dimensional structures in time series of brain electrical activity: dependence on recording region and brain state. Phys. Rev. E 64:061907, 2001.
Bronzino, J. D. The Biomedical Engineering Handbook, 3rd ed. CRC Press, Boca Raton, Sec. III, pp. 26.1–26.5, 2006.
Bruhn, J., L. E. Lehmann, et al. Shannon entropy applied to the measurement of the electroencephalographic effects of desflurane. Anesthesiology 95(1):30–35, 2001.
Coifman, R. R., and M. V. Wickerhauser. Entropy-based algorithms for best basis selection. IEEE Trans. Inf. Theory 38(2):1241–1243, 1992.
Gulbag, A., F. Temurtas, and I. Yusubov. Quantitative discrimination of the binary gas mixtures using a combinational structure of the probabilistic and multilayer neural networks. Sens. Actuat. B Chem. 131:196–204, 2008.
Guyton, A. C. Text Book of Medical Physiology. Saunders, Philadelphia, Sec.2, 968 pp, 1986.
Hagan, M. T., H. B. Demuth, and M. H. Beale. Neural Network Design. PWS Publishing, Boston, 1996.
Jang, J. S. R., C. T. Sun, and E. Mizutani. Neuro Fuzzy and Soft Computing. Prentice Hall, Englewood Cliffs, pp. 335–345, 1997.
Kannathal, N., U. R. Acharya, et al. Characterization of EEG—a comparative study. Comp. Meth. Prog. Biomed. 80:17–23, 2005. doi:10.1016/j.cmpb.2005.06.005.
Kannathal, N., M. L. Choo, et al. Entropies for detection of epilepsy in EEG. Comp. Meth. Prog. Biomed. 80:187–194, 2005. doi:10.1016/j.cmpb.2005.06.012.
Niedermeyer, E., and F. H. L. Silva (eds). Electroencephalography: Basic Principles, Clinical Applications and Related Fields, 3rd ed. Baltimore: Williams and Wilkins, pp. 661–677, 1993.
Pincus, S. M. Approximate entropy as a measure of system complexity. Proc. Natl. Acad. Sci. USA 88:2297–2301, 1991.
Rezek, I., and S. J. Roberts. Stochastic complexity measures for physiological signal analysis. IEEE Trans. BME 44(9):1186–1191, 1998.
Richard, P. B. Fast training algorithms for multi-layer neural nets. IEEE Trans. Neural Netw. 2:346–354, 1991.
Richman, J. S., and J. R. Moorman. Physiological time-series analysis using approximate entropy and sample entropy. Am. J. Physiol. Heart Circ. Physiol. 278(6):H2039–H2049, 2000.
Ricker, J. H. Differential Diagnosis in Adult Neuropsychological Assessment. Springer Publishing Company, New York, 109 pp, 2003.
Rosso, O. A., S. Blanco, J. Yordanova, et al. Wavelet entropy: a new tool for analysis of short duration brain electrical signals. J. Neurosci. Meth. 105:65–75, 2001.
Saraoglu, H. M., and B. Edin. E-Nose system for anesthetic dose level detection using artificial neural network. J. Med. Syst. 6:475–482, 2007.
Shannon, C. E. Mathematical theory of communication. Bell Syst. Tech. J. 27:379–423, 623–656, 1948.
Srinivasan, V., C. Eswaran, et al. Approximate entropy based epileptic EEG detection using artificial neural networks. IEEE Trans. Inform. Technol. Biomed. 11:288–295, 2007.
Temurtas, F., C. Tasaltin, H. Temurtas, et al. Fuzzy logic and neural network applications on the gas sensor data: concentration estimation. Lecture Notes in Computer Science, vol. 2869, pp. 179–186, 2003
Vierti, H., P. Merilainen et al. Spectral entropy, approximate entropy, complexity, fractal spectrum and bispectrum of EEG during anesthesia. In: The 5th International Conference on Memory, Awareness and Consciousness, New York, June 2001.
Author information
Authors and Affiliations
Corresponding author
Rights and permissions
About this article
Cite this article
Aydın, S., Saraoğlu, H.M. & Kara, S. Log Energy Entropy-Based EEG Classification with Multilayer Neural Networks in Seizure. Ann Biomed Eng 37, 2626–2630 (2009). https://doi.org/10.1007/s10439-009-9795-x
Received:
Accepted:
Published:
Issue Date:
DOI: https://doi.org/10.1007/s10439-009-9795-x