Abstract
REDD (Reducing Emissions from Deforestation and Forest Degradation) has been suggested as a climate change mitigation strategy that is based on the philosophy to reward countries for reducing their deforestation and forest degradation by financial benefits via the generation of carbon credits. While the potential of REDD has been widely discussed, minor attention has been drawn to the implication of uncertainties and costs associated with the estimation of carbon stock changes. To raise awareness of these issues, we conducted a simulation study for a set of countries that show high to low deforestation rates, which demonstrates that the potential to generate benefits from REDD depends highly on the magnitude of the total error while assessment costs and the price of carbon credits play a minor role. For countries with low deforestation rates REDD is obviously not an option for generating benefits as they would need to implement monitoring systems that are able to estimate carbon stock changes with a total error well below 1 %. Total errors feasible under operational monitoring systems are only sufficient to gain revenues from REDD-regimes under high deforestation rates.
Similar content being viewed by others
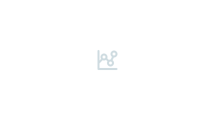
1 Background
According to UN-FAO’s Forest Resources Assessment (FRA) 2010 (FAO 2010), the world’s forests store 289 gigatonnes (Gt) of carbon in their biomass. The FRA 2010 however also shows that the destruction of forests releases 0.5 Gt of carbon annually between 1990 and 2010. The Stern-Review (Stern 2007) identified that “emissions from deforestation are very significant”. The emissions from deforestation are estimated to represent between 12 % and 20 % of global emissions (van der Werf et al. 2009; IPCC 2007), which calls for urgent activities to maintain the remaining areas of natural forests.
As part of a global climate change mitigation strategy REDD should support developing countries to take additional actions that reduce emissions from deforestation and forest degradation by mobilization and distribution of financial resources (UNFCCC 2008). A basic concern on the finance mechanisms for REDD is whether the generated financial resources should be public or private, or a mixture of both (ITTO 2009). It can be argued that short-term economic profits from deforestation and degradation offset the long-term benefits of forests (EfB 2011). REDD mechanisms aim at generating credits for maintaining current forest carbon stocks by avoiding deforestation and forest degradation. Currently, those credits are only eligible on voluntary carbon markets, but in a post-2012 climate agreement they may enter regulatory Kyoto carbon markets.
EcoSecurities (2007) presented estimates which indicate that a 50 % reduction in the world’s deforestation rate could generate between 7.6 and 45.9 Billion US$ each year depending on the monetary value per ton carbon credits. While EcoSecurities aimed at the obtainable market volumes and focussed on the potential benefits, Grieg-Gran (2008) estimated the global costs of cutting the rate of deforestation in eight countries (i.e. Bolivia, Brazil, Cameroon, DRC, Ghana, Indonesia and PNG) responsible for about 50 % of the world wide deforestation. Grieg-Gran used the simplifying assumptions that a national scheme to avoid deforestation is implemented and has 100 % additionality and no leakage, and that the alternative to deforestation is forest conservation without any exploitation of timber. She identified three cost components that need to be covered to avoid deforestation at the country level:
-
the value of the economic activity that leads to deforestation, e.g. agriculture or mining;
-
the administration, monitoring and enforcement costs for the government, and
-
an incentive element.
Grieg-Gran (2008) utilized the net present value of returns from land uses as the total costs of avoided deforestation. If foregone returns from selective logging are included the annual total cost for controlling deforestation in the eight selected countries would be between US$ 6.5 billion and US$ 8 billion.
A country that intends to participate in a future REDD mechanism has to demonstrate that it has substantial capacity of monitoring and accounting carbon emissions from forests in the future. Thus, a reliable framework for measuring, reporting and verification is urgently needed to ensure the integrity and credibility of REDD efforts in general and REDD in the post-2012 negotiation under the UNFCCC in particular (Plugge et al. 2011). While approaches for monitoring and reporting as well as financing mechanisms including the allocation of incentives have been intensively discussed (GOFC-GOLD 2010; Eliasch 2008), little attention has so far been paid to the costs and uncertainties of such operational REDD monitoring systems. In the following we compare cost and uncertainties involved in implementing a REDD monitoring system with the potential financial benefits generated by a REDD regime.
1.1 Monitoring costs
No universal inventory concept exists for REDD monitoring. A cost-efficient inventory concept needs to be adapted to the specific conditions of the inventory area and include choices on data sources utilized, in-situ assessment methods, models, sampling concepts, sampling intensity, stratification rules, time intervals for updating, or methods for quantifying errors (Köhl et al. 2006). Generally several alternative inventory concepts can be found for a specific situation, and choosing the most cost-efficient alternative is a matter of optimization (Köhl et al. 2011). Two objective functions exist in the optimization process: (1) minimizing error for given costs, or (2) minimizing cost for a desired error (Scott and Köhl 1993). The total costs of an inventory is made up of fixed and variable costs. Fixed costs do not change with the area to be monitored, e.g. expenses for developing a survey design, or for computer equipment and software development. Variable costs are expenses that change in proportion to the inventory area and the number of field plots assessed. The optimization process takes into account only variable costs.
Hardcastle and Baird (2008) studied the readiness of 25 tropical countries for monitoring forests and reporting on REDD. For each country cost estimates are provided for implementing REDD monitoring and reporting systems, the major drivers of costs being forest extent, stratification, and the appropriate choice of estimation method (Tier). They present the initial and recurrent cost separately for four alternatives:
Tier 2, Approach A: an accurate land-cover map is available, 300 sample plots are assessed in-situ, all carbon measurements are performed once at the beginning of the programme, future monitoring is focused on the assessment of human activities (activity data, AD), such as area changes by remote sensing data, and requires only minimal field work.
Tier 2, Approach B: no accurate land-cover map is available, in-situ assessments are performed when activity monitoring by remote sensing identifies locations under change, the in-situ sampling intensity is considerably lower than under Tier 2, Approach A.
Tier 3, ignoring degradation: AD und emissions per unit of the activity (emission factors, EF) are assessed as under alternative 1 (Tier 2 Approach A), but re-measurements are made in permanent in-situ sample plots (about 1/3 of the original sample locations).
Tier 3, including degradation: alternative 3 is enhanced by further stratification of forests into the two classes “intact forests” and “non-intact forests”, the number of field plots is moderately increased.
The inventory concepts applied by Hardcastle and Baird (2008) are generic rather than case-specific, as they do not result from a sound inventory design and optimization process on the individual national levels. However, they are used for an approximate comparison of cost required to implement an operational REDD monitoring and reporting scheme on the national level. Figure 1 presents the respective costs for the four alternatives over forest area. The cost per unit area decreases with increasing forest area, as the share of fixed costs in total costs decreases.
Cost estimates [US$/ha] for implementing annual forest monitoring systems in relation to forest area (source data: Hardcastle and Baird 2008) for a 5-year commitment period
1.2 Uncertainties
The principle of conservativeness, which has been reflected in several UNFCCC documents, for example in the context of afforestation and reforestation activities under the Clean Development Mechanism (CDM) (UNFCCC 2006a, b), was proposed by Grassi et al. (2008) in order to “address the potential incompleteness and high uncertainties of REDD estimates”.
According to Grassi et al. (2008), the completeness principle depends on “the processes, pools and gases that need to be reported and on the forest-related definitions”. For quantifying carbon stock changes under REDD activities both uncertainties and incompleteness need to be considered. The IPCC-Good Practice Guidance suggests in the context of the assessment of changes in soil carbon the use of the Reliable Minimum Estimate (RME) to address uncertainties (IPCC 2003). The RME was originally introduced by Dawkins (1957) as the minimum quantity to be expected with a given probability and served as a surrogate for the lower bound of a confidence interval.
However, from a statistical point of view the principle of the RME is different from the lower bound of the confidence interval that is suggested by several authors (e.g. GOFC-GOLD 2010). Where the confidence interval is used, only sampling errors are considered. The RME is based on a holistic treatment of uncertainties and includes in addition to sampling errors other error sources. Köhl et al. (2009) describe the components of the total survey error such as model and prediction errors, measurement errors, frame errors, or classification errors. In the scope of REDD, the RME is the difference between the lower error interval at the reference period (time 1) and the upper bound of the error interval at the commitment period (time 2) and can be treated as a conservative estimate that qualifies for accounting. The resulting magnitude of emission reduction is considerably smaller for an RME than for a confidence interval, which only takes sampling errors into account.
2 Methods
A rational decision about the adoption of a REDD regime is driven by the potential benefits on the one hand, and the costs for implementing an operational and sound monitoring system on the other. Comparing benefits with costs allows for calculating the break-even point (BEP), where potential benefits equal the expected monitoring costs.
The potential benefit generated by a REDD regime at the end of a commitment period, t2, is subject to the amount of carbon stock qualifying for accounting, Ct2REDD, and the prices paid per ton of CO2. Ct2REDD is calculated as the difference between the expected carbon stock under a baseline scenario without any efforts to avoid deforestation and degradation, Ct2BL, and the real carbon stock observed at time 2, Ct2real. Under the conservativeness approach (Grassi et al. 2008) uncertainties associated with the estimation of Ct2REDD need to be considered in order to obtain the RME of the carbon stock at time 2, Ct2RME (Köhl et al. 2009). Thus, the amount of reduced carbon emissions qualifying for accounting, Ĉt2REDD is obtained by:
where
- Ct2RME :
-
carbon stock at time 2 qualifying for accounting
- Ct2BL :
-
expected carbon stock at time 2 according to a baseline scenario
- ∆real :
-
proportion of real carbon stock change between time 1 and time 2, {-1,1}
- ∆BL :
-
proportion of carbon stock change between time 1 and time 2 according to the baseline, {-1,1}
- Ct1 :
-
carbon stock at time 1
- Et2 :
-
total error at time 2
Among other factors the amount of carbon stock qualifying for accounting, Ĉt2REDD, is affected by the proportion of the real change of carbon stocks between time 1 and time 2, ∆real. Human induced activities lead to either an increase or a decrease of carbon losses with respect to ∆BL. Where efforts to reduce degradation and deforestation are successful, the real change, ∆real, is smaller than the change according to the baseline, ∆BL, and an emission reduction is obtained. Where deforestation and degradation exceed prior rates, emissions are increased. ∆ real is given by
where
- ∆desired :
-
the proportional reduction of the change between time 1 and time 2 according to the baseline (i.e. negative values for ∆desired indicate a successful reduction of past emission patterns)
The possible financial earnings by means of a REDD regime result from the emission reductions, Ĉt2REDD, multiplied by the potential value of carbon credits, PC, and need to be larger than the cost for implementing and maintaining the monitoring system, M, in order to produce benefits. A breakeven-point is reached when revenues equal costs.
where
- Ĉt2REDD :
-
amount of carbon stock qualifying for accounting, incorporating uncertainties
- PC :
-
value of carbon credits
- M:
-
monitoring costs
With Equations (1) and (2) Equation (3) can be transformed (see Appendix) to show the amount of reduction, ∆desired that is needed to reach a breakeven-point between revenues and costs:
The monitoring cost can be further segregated into variable (MV) and fixed inventory costs (MF) (Scott and Köhl 1993; Wöhe et al. 2005; Hardcastle and Baird 2008). While fixed costs, such as for administration or remote sensing imagery, are design independent, the variable costs vary with sample size, as shown in formula (5).
where
- MV :
-
Variable inventory costs
- MF :
-
Fixed inventory costs
- A:
-
Forest area [ha]
- Mha :
-
Assessment costs per hectare forest
Under the constraint that no degradation and only deforestation takes place and thereby the carbon stock per unit area remains constant, i.e. \( {\overline {\text{C}}_{{{\text{t}}2}}} = {\overline {\text{C}}_{{{\text{t}}1}}} \), and with
where
\( {\overline {\text{C}}_{{{\text{t}}2}}} \) is the carbon stock per hectare at time 2
rearranging Eq. (6) produces
Equations (5) and (7) were used to transform Eq. (4):
2.1 Simulation study
To show the effect of the inclusion of uncertainties and monitoring costs in REDD estimates, a simulation study was conducted. The rate of deforestation between 2000 and 2010 was utilised to construct the baseline and thereby predict the carbon stock at the end of the commitment period under a business-as-usual (BAU) development. The objective function utilized was the amount of reduction of deforestation with respect to the baseline scenario needed for reaching a break-even point between revenues gained from a REDD scheme and the costs of the underlying monitoring system.
FAO’s Global Forest Resources Assessment (FAO 2010) was utilized to select five countries, which show small to large forest areas and low (−0.12 %) to high (−23.36 %) deforestation rates. Table 1 presents the corresponding data as given in FRA 2010 on forest area and carbon stock, which were used to calculate the proportional changes between time 1 and time 2 (reference period = 10 years) according to the baseline, ∆BL.
For simulating the impacts of monitoring costs and uncertainties realistic ranges for total errors, Et2, and per hectare monitoring costs, Mha, had to be defined. For total errors, Et2, a range between 1 % and 10 % (1 %, 2 %, 5 %, 10 %) was chosen, for the variable monitoring costs, Mha, a range between 5US$/ha to 0.01US$/ha (5US$/ha, 1US$/ha, 0.1US$/ha, 0.01US$/ha). Fixed monitoring costs, MF, were set to 100,000US$ and include inter alia the costs for remote sensing imagery. The total cost of the monitoring system was calculated by the product of the national forest area and the respective per ha assessment costs plus the fixed monitoring costs. Under these conditions the fixed costs are well below 2 % of the total costs, if the per ha assessment costs, Mha, are 1US$/ha or higher. For assessment costs of 0.1US$/ha they vary between 1.1 % (Indonesia) to 16.8 % (Ghana) and 9.6 % (Indonesia) and 66 % (Ghana) for 0.01US$/ha. The price paid per ton of carbon credit, PC, was set to 10 US$/tCO2.
3 Results
For each of the five selected countries Eq. (8) was applied using the figures presented in Table 1 and the value ranges for errors and costs given above in order to predict the associated proportional reduction of the carbon stock change between time 1 and time 2 with respect to the baseline, ∆desired, that is needed to reach a break-even point between assessment costs and revenues from REDD. The results for ∆desired are presented in Table 2 and show under the given cost and total error scenarios the reduction of past deforestation rates (∆BL) that is needed to just cover the assessment costs by revenues from reduced deforestation. Under given cost and total errors gains are produced where ∆desired is larger than the respective value shown in Table 2.
Where table cells in Table 2 show values for ∆desired larger than 100 % a country cannot realize benefits from reduced deforestation only but would additionally need to increase its forest area in the commitment period in order to at least cover the assessment costs. The results given in Table 2 show that Ghana and Cameroon as the two countries with the highest deforestation rates (ΔBL = −23.36 % and −11.05 % respectively) can benefit in all error/cost constellations from REDD without increasing its forest area. While for total errors of 1 % to 2 % even a moderate reduction of the deforestation with respect to the baseline would yield benefits from REDD for these two countries, the deforestation of Cameroon would need to be almost halted for a 10 % total error.
Indonesia, with a high forest area and a medium deforestation rate (ΔBL = −5.27 %), is in a position to benefit from a REDD scheme only under the first two simulated error scenarios (i.e. 1 % and 2 %). With a total error of 5 % Indonesia would need to nearly halt its deforestation, while for a total error of 10 % the forest area would have to be doubled during the commitment period (resulting in total forest area values of 199.71 % to 201.69 % relative to the beginning of the commitment period) to reach a breakeven-point of costs and revenues.
The outcomes of the simulation study for Colombia, a country with high forest area and low deforestation rate (ΔBL = −1.67 %), show that only under a scenario where the deforestation rate is more than halved and the total error is well below 2 % benefits from REDD can be achieved without increasing the forest area.
Suriname as the country with the lowest deforestation rate in our simulation study (ΔBL = −0.12 %) is under none of the simulated error and cost scenarios in a position to benefit from a REDD scheme. On the contrary Suriname would need to increase its forest area substantially (i.e. at least by 727.34 %) to reach a breakeven-point of costs and revenues. This however is not even hypothetically possible, as this would exceed the total land area of Suriname itself.
On the whole, Table 2 obviously demonstrates two major findings on the potential to generate benefits from REDD. One is case specific for this study, as we have chosen a simple business-as-usual baseline scenario. Under this scenario past deforestation rates have a strong effect on the potential to generate benefits from REDD. The second finding is a more generic one. The impacts of the total error are much higher than the influence of the assessment costs per hectare. Thus countries in the readiness phase of REDD need to put uttermost attention and efforts in developing assessments schemes that minimize total errors and produce reliable results.
Benefits from REDD can only be generated where the RME of the carbon stock at time 2, Ct2RME, is larger than the carbon stock defined by the baseline, Ct2BL. Table 3 presents the threshold values for total errors at which Ct2RME equals Ct2BL under the assumption that the deforestation was halved (Δdesired = 50 %). Countries with low deforestation rates (i.e. Colombia and Suriname) would need to implement monitoring systems that are able to estimate carbon stock changes with a total error well below 1 %. Only under high deforestation rates total errors feasible under operational monitoring systems are sufficient to cover the assessment costs. As the thresholds for Ct2RME refer solely to the consideration of errors, they elude influence by either assessment costs or carbon prices.
The incorporation of assessment costs, M, into this calculation would decrease the threshold values for total errors. The limited influence of the value of carbon credits, PC, on the potential benefits generated from REDD can be seen in Fig. 2, where thresholds for total errors of each country are presented as a function of PC, and assessment cost as shown in formula (9). It becomes evident that the more the values of Ct2RME and Ct2BL converge (however still Ct2RME > Ct2BL), the influence of the value of carbon credits on a possible compensation of assessment costs vanishes.
Equations (1) and (7) were used to transform Eq. (9):
For the calculations fixed costs, MF, were set to 100.000 US$ and variable costs, Mha, to 0.1 US$/ha. Figure 2 presents the total error percentages over carbon prices. For higher values of carbon credits, PC, a larger error can be accepted for estimating the carbon stock at time 2 in order to balance the expenses for assessments and the revenues by carbon credits. However, an increase of PC from 0.01$/tCO2 to 10$/tCO2 results in a situation where any of the threshold values presented in Table 3 are reached. Figure 2 clearly shows that under the above described preconditions for each country an asymptote for the Eq. 10 exists. This means that for Eq. 10 there is no significant effect of PC for a value of carbon credits higher than 1$/tCO2. Thus, increasing the value of carbon credits can only show a restricted contribution to a possible generation of benefits from REDD, as not values of carbon credits and assessment costs but the total errors of carbon stock assessment are the limiting factors.
It is still arguable which magnitude of total errors can be reached in REDD monitoring systems. Germany, for example, has a sophisticated national forest inventory and reports a 1.4 % sampling error for estimating the growing stock (BMELV 2011). Gertner and Köhl (1992) showed for the example of the Swiss national forest inventory that the inclusion of non-sampling errors and bias can inflate the total error of growing stock estimates substantially. According to Waggoner (2009) a 1 % level of total error for the estimation of carbon stock changes will be extremely difficult to be met.
4 Discussion
Generally, the potential to generate benefits from REDD depends on the deforestation rate of the respective country, the assessment costs and the uncertainties associated with the estimation of the carbon stock at the end of the reference period, time 2. The simulation study conducted showed that countries with already low deforestation rates are not in an easy position to gain benefits from REDD. On the contrary, those countries would be forced to increase their forest area in order to meet the reduction goals when the total error of the estimate of the carbon stock at time 2 is taken into account.
However, it was demonstrated that in fact the potential to generate benefits from REDD depends highly on the magnitude of the total error, while assessment costs and the values of carbon credits play a minor role. Nevertheless, the influence of assessment costs should not be peculated. There are a number of possibilities to optimize the cost-effectiveness of an assessment scheme (Köhl et al. 2011). Furthermore there are numerous case studies of indigenous participation in scientific data collection projects which are helpful in reducing assessment costs with the application of Participatory Forest Carbon Assessments (Galloway McLean 2010).
However, under the preconditions set for this simulation study it becomes obvious that for countries with low deforestation rates (i.e. Colombia and Suriname) REDD is not an option for generating benefits as they would need to implement monitoring systems that are able to estimate carbon stock changes with a total error well below 1 %. By larger error levels no RME for the carbon stock at the end of the reference period (time 2) above the carbon stock level as indicated by the baseline can be achieved. As the underlying functional relationships depend only on possible sources of error, no improvements can be achieved by reducing assessment costs or realizing higher values of carbon credits. Total errors feasible under operational monitoring systems are sufficient to gain revenues from REDD-regimes only under high deforestation rates.
5 Conclusions
Uncertainties associated with the quantification of carbon stocks and carbon stock changes exert a dominant influence on the generation of carbon credits under a REDD regime. The operational implementation of REDD as a global climate change mitigation strategy renders mandatory regulations for the assessment and accounting of errors necessary. Otherwise countries would be discriminated against on accord of sound monitoring and reporting methods.
When the principle of conservativeness is not reflected in REDD accounting regulations, countries would be well advised to apply imprecise and inaccurate monitoring systems.
Countries in the readiness phase of REDD need to put uttermost attention and efforts in developing assessments schemes that minimize total errors and produce reliable results. This holds especially true for countries that have already reached a situation where deforestation rates are low. The need for capacity building and the development of cost-efficient monitoring systems that produce estimates with high reliability is crucial for the successful implementation of REDD.
Countries showing low deforestation rates are discriminated against the generation of REDD benefits; they meet improvements with respect to national baselines only when their monitoring systems are able to produce results with extremely low total errors. In order not to exclude those countries per se from REDD, several alternatives for establishing national baselines have been proposed. Among those alternatives are:
-
the discrimination between countries with high and low deforestation rates and the introduction of a “global” baseline rate for the latter (Mollicone et al. 2007),
-
the allocation of credits to an individual country by applying a formula that combines a measure of individual country performance against their own historic emissions’ baseline, and performance against a global emissions’ baseline (Strassburg et al. 2009),
-
the allocation of credits to countries as a function of both reduced emissions from deforestation - as compared with historical rate-, and as dividends for maintaining carbon stocks–as a proportion of global forest carbon stocks (Cattaneo 2008; Cattaneo 2010),
-
a separate system not based on carbon stock changes but rewarding conservation activities or sustainable management of forests by evaluating policies and measures undertaken and achieved (Meridian Institute 2011).
Those alternatives have to be examined against the background of uncertainties in order to provide comparable and equitable accounting schemes and to avoid windfall profits for countries with unsophisticated assessment systems.
References
BMELV (2011) Bundeswaldinventur—the National Forest Inventory. http://www.bundeswaldinventur.de/enid/6a58daffd51c0d46d88bfb4ca088fd05.0/75.html. Accessed 21 Nov 2011
Cattaneo A (2008) How to distribute REDD funds across countries? a stock-flow mechanism. Submission to the United Nations Framework Convention on Climate Change regarding AWG–LCA (FCCC/AWGLCA/2008/L.7)
Cattaneo A (2010) Incentives to reduce emissions from deforestation. A stockflow approach with target reductions. In: Bosetti V, Lubowski R (eds) Deforestation and climate change. Reducing carbon emissions from deforestation and forest degradation; [International Workshop on REDD; November 2008]. Elgar, Cheltenham
Dawkins HC (1957) Some results of stratified random sampling of tropical high-forest. Seventh British Commonwealth Forestry Conference Item 7 (iii), Oxford
EcoSecurities (2007) Policy brief:. REDD policy scenarios and carbon markets. A report commissioned by the Government of Indonesia and the World Bank, Oxford, UK; Jakarta, Indonesia
EfB (2011) What are the benefits of forests and the consequences of deforestation? http://www.environmentforbeginners.com/content/view/52/49/
Eliasch J (2008) Climate change. Financing global forests. the Eliasch review. Earthscan, London
FAO (2010) Global forest resources assessment 2010: main report. FAO forestry paper, vol 163. Food and Agriculture Organization of the United Nations, Rome
Galloway McLean K (2010) Advance guard: climate change impacts, adaptation, mitigation and indigenous peoples. A Compendium of Case Studies, Darwin
Gertner G, Köhl M (1992) An assessment of some nonsampling errors in a national survey using an error budget. For Sci 38(3):525–538, 14
GOFC-GOLD (2010) A sourcebook of methods and procedures for monitoring and reporting anthropogenic greenhouse gas emissions and removals caused by deforestation, gains and losses of carbon stocks in forests remaining forests, and forestation. a sourcebook of methods and procedures for monitoring, measuring and reporting. GOFC-GOLD Report version COP16-1. GOFC-GOLD Project Office, Natural Resources Canada, Alberta, Canada, Alberta, Canada
Grassi G, et al. (2008) Applying the conservativeness principle to REDD to deal with the uncertainties of the estimates. Environ Res Lett (3)
Grieg-Gran M (2008) The cost of avoiding deforestation. Update of the Report prepared for the Stern Review of the Economics of Climate Change
Hardcastle PD, Baird D (2008) Capability and cost assessment of the major forest nations to measure and monitor their forest carbon. Report prepared for the Office of Climate Change, Penicuick, UK
IPCC (2003) Good practice guidance for land use, land-use change and forestry. IPCC National Greenhouse Gas Inventories Programme. Institute for Global Environmental Strategies (IGES), Hayama
IPCC (2007) Climate Change 2007: Impacts, Adaptation and Vulnerability. Contribution of Working Group II to the Fourth Assessment Report of the Intergovernmental Panel on Climate Change. In: Parry ML, Canziani OF, Palutikof P, van der Linden PJ, Hanson CE, (eds) Cambridge University Press, Cambridge, UK, p 976
ITTO (2009) Tropical timber market report, 14th edn
Köhl M, Magnussen S, Marchetti M (2006) Sampling methods, remote sensing and GIS multiresource forest inventory. Springer-11642/Dig. Serial]. Springer, Berlin
Köhl M, Baldauf T, Plugge D, Krug J (2009) Reduced emissions from deforestation and forest degradation (REDD): a climate change mitigation strategy on a critical track. Carbon Balance Manag 4:10. doi:10.1186/1750-0680-4-10
Köhl M, Lister A, Scott C, Baldauf T, Plugge D (2011) Implications of sampling design and sample size for national carbon accounting systems. Carbon Balance Manag 6(1):10
Meridian Institute (2011) Modalities for REDD+ reference levels:. Technical and procedural issues. Prepared for the Government of Norway, Norway
Mollicone D, Achard F, Federici S, Eva H, Grassi G, Belward A, Raes F, Seufert G, Stibig H, Matteucci G, Schulze E (2007) An incentive mechanism for reducing emissions from conversion of intact and non-intact forests. Clim Chang 83:477–493. doi:10.1007/s10584-006-9231-2
Plugge D, Baldauf T, Köhl M (2011) Reduced emissions from deforestation and forest degradation (REDD): why a robust and transparent monitoring, reporting and verification (MRV) system is mandatory. In: Blanco J, Kheradmand H (eds) Climate change–research and technology for adaptation and mitigation. InTech, Rijeka, pp 155–170
Scott C, Köhl M (1993) A method for comparing sampling design alternatives for extensive inventories. Mitteilungen der Eidgenössischen Forschungsanstalt für Wald, Schnee und Landschaft, vol 68. Eidg. Forschungsanstalt für Wald, Schnee und Landschaft, Birmensdorf
Stern N (2007) The economics of climate change. The Stern review. Cambridge University Press, Cambridge
Strassburg B, Turner RK, Fisher B, Schaeffer R, Lovett A (2009) Reducing emissions from deforestation—the “combined incentives” mechanism and empirical simulations. Traditional Peoples and Climate Change. Glob Environ Chang 19(2):265–278. doi:10.1016/j.gloenvcha.2008.11.004
UNFCCC (2006a) Good practice guidance and adjustments under Article 5, paragraph 2, of the Kyoto Protocol. FCCC/KP/CMP/2005/8/Add.3 Decision 20/CMP.1
UNFCCC (2006b) Modalities and procedures for afforestation and reforestation project activities under the clean development mechanism in the first commitment period of the Kyoto Protocol. Decision 5/CMP.1
UNFCCC (2008) Reducing emissions from deforestation in developing countries: approaches to stimulate action. FCCC/SBSTA/2008/L.12
van der Werf GR, Morton DC, DeFries RS, Olivier JGJ, Kasibhatla PS, Jackson RB, Collatz GJ, Randerson JT (2009) CO2 emissions from forest loss. Nat Geosci 2(11):737–738
Waggoner PE (2009) Forest inventories. Discrepancies and uncertainties. DISCUSSION PAPER. RFF, Washington, USA
Wöhe G, Kaiser H, Döring, U (2005) Übungsbuch zur Einführung in die allgemeine Betriebswirtschaftslehre. 11th edn. Vahlen, München
Acknowledgments
We are grateful to three anonymous reviewers, which helped to improve the quality of the text.
Open Access
This article is distributed under the terms of the Creative Commons Attribution License which permits any use, distribution, and reproduction in any medium, provided the original author(s) and the source are credited.
Author information
Authors and Affiliations
Corresponding author
Appendix
Appendix
Proportional reduction of change between time 1 and time 2 according to the baseline, ∆desired
∆desired under cost constraints
Et2 Breakeven
Rights and permissions
Open Access This article is distributed under the terms of the Creative Commons Attribution 2.0 International License (https://creativecommons.org/licenses/by/2.0), which permits unrestricted use, distribution, and reproduction in any medium, provided the original work is properly cited.
About this article
Cite this article
Plugge, D., Baldauf, T. & Köhl, M. The global climate change mitigation strategy REDD: monitoring costs and uncertainties jeopardize economic benefits. Climatic Change 119, 247–259 (2013). https://doi.org/10.1007/s10584-012-0524-3
Received:
Accepted:
Published:
Issue Date:
DOI: https://doi.org/10.1007/s10584-012-0524-3