Abstract
Seventeen tea accessions belonging to Chinese (Camellia sinensis), Assamic (C. sinensis var. assamica), and Shan tea (C. sinensis var. pubilimba) groups, which are either commercially planted or new promising tea germplasm, were morphologically described at Phu Tho province (Viet Nam) and assessed for their diversity. Fourteen phyllometric parameters were qualitatively and quantitatively investigated using digital image analysis. The accessions were then discriminated by a dedicated artificial neural network for univocal plant identification and a hierarchical cluster analysis was performed in order to build a dendrogram reporting the relationships among them. Results proved the diversity of investigated tea morphotypes from Phu Tho province based on a morphological screening. More, the artificial neural network was able to perform a correct identification for almost all the accessions using simple dedicated instruments.



Similar content being viewed by others
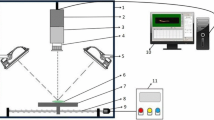
Abbreviations
- ANN:
-
Artificial neural network
- BPNN:
-
Back-propagation neural network
References
Chang HT (1981) A taxonomy of the genus Camellia. Acta Sientiarum Naturalium Universitatis Sunyatseni 1–124
Chang HT (1984) A revision of the tea resource plants. Acta Sientiarum Naturalium Universitatis Sunyatseni 1–12
Chen L, Yamaguchi S (2005) RAPD markers for discriminating tea germplasm at the inter-specific level in China. Plant Breed 124:404–409. doi:10.1111/j.1439-0523.2005.01100.x
Chen L, Yao MZ, Yang YJ, Yu YJ (2006a) Collection, conservation, evaluation and utilization of tea genetic resources (Camellia spp.) in China. Floriculture, ornamental and plant biotechnology: advances and topical issues. Glob Sci Books UK 1:578–583
Chen L, Yao MZ, Zhao LP, Wang XC (2006b) Recent research progresses on molecular biology of tea plant (Camellia sinensis). Floriculture, ornamental and plant biotechnology: advances and topical issues. Glob Sci Books UK 4:425–436
Clark JY (2004) Identification of botanical specimens using artificial neural networks. Proceedings of the 2004 IEEE symposium on computational intelligence in bioinformatics and computational biology, La Jolla, USA, 7th–8th October 2004, pp 87–94
Kaundun SS, Matsumoto S (2002) Heterologous nuclear and chloroplast microsatellite amplification and variation in tea, Camellia sinensis. Genome 45:1041–1048. doi:10.1139/g02-070
Kaundun SS, Zhyvoloup A, Park YG (2000) Evaluation of the genetic diversity among elite tea (Camellia sinensis var. sinensis) genotypes using RAPD markers. Euphytica 115:7–16. doi:10.1023/A:1003939120048
Lu CY, Liu WH, Li MJ (1992) Relationship between the evolutionary relatives and the variation of esterase isozymes in tea plant. J Tea Sci 12:15–20
Mancuso S (1999) Fractal geometry-based image analysis of grapevine leaves using the box counting algorithm. Vitis 38:97–100
Mancuso S, Nicese FP (1999) Identifying olive (Olea europaea L.) cultivars using artificial neural networks. J Am Soc Hortic Sci 124:527–531
Mancuso S, Ferrini F, Nicese FP (1999) Chestnut (Castanea sativa L.) genotype identification: an artificial neural network approach. J Hortic Sci Biotechnol 74:777–784
Mariño SI, Tressens SG (2001) Artificial neural networks application in the identification of three species of Rollinia (Annonaceae). Ann Bot Fenn 38:215–224
Mondal TK (2002) Assessment of genetic diversity of tea (Camellia sinensis (L.) O. Kuntze) by inter-simple sequence repeat polymerase chain reaction. Euphytica 128:307–315. doi:10.1023/A:1021212419811
Morgan A, Boddy L, Mordue JEM, Morris CW (1998) Evaluation of artificial neural networks for fungal identification employing morphometric data from spores of Pestalotiopsis species. Mycol Res 102:975–984. doi:10.1017/S0953756297005947
Mugnai S, Pandolfi C, Azzarello E, Masi E, Mancuso S (2008) Camellia japonica L. genotypes identified by an artificial neural network based on phyllometric and fractal parameters. Plant Syst Evol 270:95–108. doi:10.1007/s00606-007-0601-7
Pandolfi C, Mugnai S, Azzarello E, Masi E, Mancuso S (2006) Fractal geometry and neural networks for the identification and characterization of ornamental plants. In: Teixeira da Silva J (ed) Floriculture, ornamental and plant biotechnology: advances and topical issues, vol IV. GlobalScience Books, Kyoto, pp 213–225
Paul S, Wachira FN, Powell W, Waugh R (1997) Diversity and genetic differentiation among populations of Indian and Kenyan tea (Camellia sinensis (L.) O. Kuntze) revealed by AFLP markers. Theor Appl Genet 94:255–263. doi:10.1007/s001220050408
Rumelhart DE, Hinton GE, Williams RJ (1986) Learning representations by back propagating errors. Nature 323:533–536. doi:10.1038/323533a0
Saravanan M, Maria John KM, Raj Kumar R, Pius PK, Sasikumar R (2005) Genetic diversity of UPASI tea cones (Camellia sinensis (L.) O. Kuntze) on the basis of total catechins and their fractions. Phytochemistry 66:561–565. doi:10.1016/j.phytochem.2004.06.024
Sealy J (1958) A revision of the genus Camellia. Royal Hort Soc, London
Ueno S, Yoshimaru H, Tomaru N, Yamamoto S (1999) Development and characterization of microsatellite markers in Camellia japonica L. Mol Ecol 8:335–336. doi:10.1046/j.1365-294X.1999.00534.x
Wight W (1962) Tea classification revised. Curr Sci 31:298–299
Willson KC, Clifford MN (1992) Tea: cultivation to consumption. Chapman & Hall, London
Yamamoto T, Juneja LR, Chu DC, Kim M (1997) Chemistry and application of green tea. CRC Press, Boca Raton, FL
Yao MZ, Chen L, Liang YR (2008) Genetic diversity among tea cultivars from China, Japan and Kenya revealed by ISSR markers and its implication for parental selection in tea breeding programmes. Plant Breed 127:166–172. doi:10.1111/j.1439-0523.2007.01448.x
Zurada JM, Malinowski A (1994) Multilayer perceptron networks: selected aspects of training optimization. Appl Math Comp Sci 4:281–307
Acknowledgments
The Authors would like to thank Mr. Nguyen Huu La, Head of the Department for Genetic Resources of the Tea Research Institute of Viet Nam (TRI), and Mr. Nguyen Van Tao, Director of for the Tea Research Institute of Viet Nam (TRI), for their technical support and assistance.
Author information
Authors and Affiliations
Corresponding author
Rights and permissions
About this article
Cite this article
Pandolfi, C., Mugnai, S., Azzarello, E. et al. Artificial neural networks as a tool for plant identification: a case study on Vietnamese tea accessions. Euphytica 166, 411–421 (2009). https://doi.org/10.1007/s10681-008-9828-9
Received:
Accepted:
Published:
Issue Date:
DOI: https://doi.org/10.1007/s10681-008-9828-9