Abstract
Temporal mass variations in the Earth system, which can be detected from the Gravity Recovery and Climate Experiment (GRACE) mission data, cause temporal variations of geoid heights. The main objective of this contribution is to analyze temporal variations of geoid heights over the area of Poland using global geopotential models (GGMs) developed on the basis of GRACE mission data. Time series of geoid height variations were calculated for the chosen subareas of the aforementioned area using those GGMs. Thereafter, these variations were analyzed using two different methods. On the basis of the analysis results, models of temporal geoid height variations were developed and discussed. The possibility of prediction of geoid height variations using GRACE mission data over the area of Poland was also investigated. The main findings reveal that the geoid height over the area of Poland vary within 1.1 cm which should be considered when defining the geoid model of 1 cm accuracy for this area.
Similar content being viewed by others
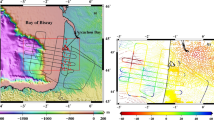
Introduction
Knowledge of a precise geoid represented by the equipotential surface at the mean sea level of a hypothetical ocean at rest is one of the fundamental tasks of geodesy. The precise geoid model serves first of all as a reference surface for heights, but it is also needed for the transformation between geometrical ellipsoidal heights obtained from the Global Navigation Satellite System (GNSS) measurements and gravity-related heights, e.g., orthometric and normal heights, determined with the use of spirit levelling. It is also required in many Earth’s science disciplines, for example, in the solid Earth geophysics to interpret and understand the Earth’s interior mass distribution. In oceanography, the precise geoid model is needed to study the sea surface topography and its dynamics, as well as the ocean circulation, which is responsible for a large part of the global heat and energy transport, and thus plays a crucial role in climate regulation.
In the recent two decades, a significant progress has been achieved in the determination of geoid models, as well as its temporal variations. In particular, the three dedicated gravity field space missions: CHAMP (CHAllenging Minisatellite Payload, 15 July 2000; Reigber et al. 2002), GRACE (Gravity Recovery And Climate Experiment, 17 March 2002; Tapley et al. 2004), and GOCE (Gravity field and steady-state Ocean Circulation Explorer, 17 March 2009; Floberghagen et al. 2011) have considerably improved the knowledge concerning the Earth’s gravity field and its temporal variations. The modelling of the static geoid at the accuracy level of 1 cm has become achievable in global and regional/local scales (e.g., Tscherning et al. 2000; Krynski and Lyszkowicz 2006). Currently, the modelling of regional geoid/quasi-geoid with sub-centimetre accuracy is considered as one of the activities of the Commission 2—Gravity Field of the International Association of Geodesy (IAG). The Joint Study Group 0.15 (JSG 0.15) concerning the regional geoid/quasi-geoid modelling—Theoretical framework for the sub-centimetre accuracy at the Intercommission Committee on Theory (ICCT) has been established for the period from 2015 to 2019 (see Drewes et al. 2016). However, since the Earth is a dynamic planet (cf. SESWG 2002), temporal variations of the geoid remain one of problems that should be considered for scientific purposes as well as for high precision applications.
Since the launch of GRACE mission, temporal mass variations in the Earth system have become detectable with unprecedented accuracy and spatial resolution (Tapley et al. 2004). This extremely successful mission is expected to be in operation until 2018 (see Tapley et al. 2015). Information on the Earth system obtained from GRACE mission is of great importance for Earth sciences. It indicates the need for the continuation of GRACE-type satellite missions which could provide a long-term continuous information on mass transport in the Earth system. The first one which will overlap with GRACE mission is GRACE Follow-on (GRACE-FO) mission that has been scheduled for launch in August 2017 (e.g., Flechtner et al. 2016). During the past years, many investigations (e.g., Tapley et al. 2004; Chambers 2006; Swenson and Wahr 2007; Luthcke et al. 2013; Krynski et al. 2014; Wu and Heflin 2015; Guo et al. 2016) concerning the determination of temporal mass variations in the Earth system using GRACE data have been conducted worldwide. For temporal geoid height variations, Rangelova (2007) combined GRACE data with GNSS, tide gauge/altimetry, and absolute gravimetry data to develop a dynamic geoid model for Canada. Rangelova and Sideris (2008) estimated the secular geoid changes in North America using GRACE and terrestrial data. The resulting dynamic geoid obtained accordingly to these studies was implemented as a vertical datum for orthometric heights in Canada (cf. Rangelova et al. 2010). Krynski et al. (2014) conducted a research for analyzing variations of the Earth’s gravity field over the whole area of Europe using release 04 (RL04) GRACE-based global geopotential models (GGMs). They showed that amplitudes of geoid height variations for the area of Central Europe reach up to 7 mm.
In this contribution, the focus is put on the latest release, i.e., release 05 (RL05), GRACE-based GGMs and temporal variations of geoid heights over a smaller area [higher spatial resolution than in Krynski et al. (2014)]. The area of Poland, bounded by parallels of 49°N and 55°N and meridians of 14°E and 24°E, has been chosen as a study area (Fig. 1). In this area, during the last two decades, an intensive research has been conducted in the field of geoid modelling (for more details, see Krynski 2007). Currently, the estimated fit of the static quasi-geoid model developed over this area to different sets of GNSS/levelling data ranges from 1.4 to 2.2 cm in terms of the standard deviation of differences (e.g., Szelachowska and Krynski 2014). The main objective of this paper is to analyze and model temporal variations of geoid heights in the area of Poland using RL05 GRACE-based GGMs. It concerns also the possibility of predicting temporal geoid height variations from GRACE mission data over the aforementioned area.
Data used
In this study, a preparatory investigation was conducted to select suitable GRACE data and a filtering method. The focus was put on monthly geoid height variations computed from GRACE-based GGMs for the period between April 2002 and March 2016 (cf. “Computation of temporal geoid height variations”). The monthly RL05 GRACE-based GGMs developed by GFZ (GeoForschungsZentrum), CSR (Center for Space Research), and JPL (Jet Propulsion Laboratory) (cf. Bettadpur 2012; Dahle et al. 2014; Watkins and Yuan 2014), were considered.
To reduce noise, which is especially strong at higher spherical harmonic degrees of these GGMs, decorrelation (DDK) filters and Gaussian filters (Wahr et al. 1998; Kusche 2007; Kusche et al. 2009) were investigated. For the area of Poland, the DDK1 and DDK2 filters reduce the noise sufficiently, but they remove all signals beyond d/o 30–50. On the other hand, geoid height variations obtained after applying the DDK7 and DDK8 filters as well as Gaussian filters with radii 300, 500, and 700 km are dominated by a significant noise. The DDK5 and DDK6 filters seem not sufficient to reduce the noise included in some GRACE-based GGMs. The DDK3 and DDK4 filters are a compromise between reducing noise and keeping signal over the investigated area. The GGMs after applying the DDK3 and DDK4 filters contain temporal geoid height variations signal up to d/o 60–70.
Temporal geoid height variations obtained from RL05 GRACE-based GGMs were then evaluated using the WGHM (WaterGAP (Water—a Global Assessment and Prognosis) Global Hydrological Model; Döll et al. 2003). The standard deviations of the differences between temporal geoid heights variations obtained from GGMs provided by CSR, GFZ, and JPL and the corresponding ones obtained from the WGHM were estimated. They are compatible within ±0.2 mm. This may reveal that differences between geoid height variations obtained from GGMs provided by CSR, GFZ, and JPL are negligible for the investigated area.
Overall, GFZ RL05 GRACE-based GGMs filtered using the DDK3 filter and truncated at d/o 60 were selected for the computation of temporal geoid height variations in this study. Considering spatial resolutions of those models, the area of Poland was divided into four subareas of 3º × 5º (Fig. 1) for which temporal geoid height variations were determined separately.
Computation of temporal geoid height variations
The geoid heights N were computed from the model of maximum degree 60 as follows (Torge and Müller 2012):
where r is the distance to the geocentre, φ and λ are the geographical latitude and longitude, respectively, θ is geographical co-latitude (90 − φ), GM is the product of the Newtonian gravitational constant G and the Earth’s mass M, a is the semi-major axis of the reference ellipsoid, \(\overline{P}_{lm}\) is the fully normalised Legendre polynomial of degree l and order m, \(\Delta \overline{C}_{lm}^{\text{DDK}}\) and \(\Delta \overline{S}_{{_{lm} }}^{\text{DDK}}\) are residual fully normalised spherical harmonic coefficients, being defined as differences between the actual and the normal gravity field (Torge and Müller 2012) and filtered using the DDK3 filter (cf. Kusche 2007; Kusche et al. 2009), and γ is the normal gravity.
The ICGEM calculation service (cf. http://icgem.gfz-potsdam.de/ICGEM/ICGEM.html) was used in the computation of geoid heights expressed in Eq. (1), at the center point for each of the aforementioned subareas (Fig. 1). Thereafter, temporal variations of geoid heights ΔN were calculated as follows:
where i denotes the month and N mean is the mean value obtained from the time series of N i . In addition, averages of monthly geoid height variations ΔN (avg) i were calculated as follows:
The differences between ΔN (avg) i and temporal variations of geoid heights computed at the central point of the investigated area using GFZ RL05 GRACE-based GGMs truncated at d/o 30 do not exceed ±0.25 mm. Thus, ΔN (avg) i can be considered as representing the low spectral resolution, i.e., d/o 30, of temporal variations of geoid heights over the whole investigated area. The differences between ΔN i for subareas 1, 2, 3, 4, and ΔN (avg) i were also computed. Their statistics are given in Table 1.
The resulting time series of geoid height variations and their spatial representation are shown in Figs. 2 and 3. They exhibit a distinctive seasonal geoid height variation pattern with maximum values at the beginning of spring and minimum values at the end of the summer. This seasonal pattern is consistent with the hydrological cycle in the investigated area (e.g., Krynski et al. 2014). The results presented in Figs. 2 and 3 also illustrate that changes in geoid height can be observed not only from epoch to epoch, but they are different for subareas at the same epoch. They reveal that geoid height variation differences from epoch to epoch at the same subarea can reach 10 and 2 mm between two subareas for the same epoch. Figure 3 depicts that GFZ RL05 GRACE-based GGMs without gaps are available for the period from January 2004 to December 2010; for the remaining period, some gaps occur. The statistics presented in Table 1 additionally shows that the dispersion and the standard deviation of differences between ΔN i for each subarea and ΔN (avg) i reach the level of 2 and 0.5 mm, respectively. This may justify the need for the analysis and modelling ΔN over the area of Poland divided into four subareas. It indicates that more geoid height variation signal can be detected when reducing the size of the computational area.
Analysis of temporal geoid height variations
The temporal geoid height variations obtained for four subareas ΔN i as well as their average ΔN (avg) i were analyzed. To avoid gaps in ΔN time series specified in “Computation of temporal geoid height variations”, data from the period between January 2004 and December 2010 were chosen for the analysis. The analyses were performed using two different methods: (1) the spectral analysis method and (2) the seasonal decomposition method. The basics of these methods are given in “The basics of the spectral analysis method” and “The basics of the seasonal decomposition method”. The implementation of these methods for the analysis of temporal geoid height variations over the area of Poland as well as their outcomes are given in “Analysis of temporal geoid height variations using spectral analysis method and the seasonal decomposition method”.
The basics of the spectral analysis method
The spectral analysis method is based on the Fourier theorem stating that a function f(t), which obeys Dirichlet’s conditions, can be represented as [e.g., Eq. (2) in Likkason 2011; p. 30]:
where a 0, a n , and b n are Fourier coefficients defined as
The a 0, a n , and b n can be considered as multiple linear regression coefficients that reflect the degree to which the respective cosine and sine functions are correlated with the data. These coefficients are used to compute periodogram values P [see Eq. (13.1.4) in Wei 2006; p. 290]:
where M is the number of elements in the time series.
The basics of the seasonal decomposition method
The general mathematical representation of the seasonal decomposition can be written as [see Eq. (3.1) in Makridakis et al. 1998; p. 84]
where Y t is the time series value at t, and S t , T t , and E t are the seasonal component, the trend-cycle component, and the irregular component at t, respectively.
A common approach is to assume Eq. (7) as the additive form [see Eq. (3.16) in Makridakis et al. 1998; p. 106]:
It can alternatively be presented in the multiplicative form [see Eq. (3.17) in Makridakis et al. 1998; p. 106]:
Analysis of temporal geoid height variations using the spectral analysis method and the seasonal decomposition method
In the spectral analysis method, ∆N time series are presented as follows:
where S F i is a seasonal component obtained on the basis of the Fourier theorem, T F i presents a trend component obtained using a suitable mathematical model, and ε i is an unmodelled component.
The implementation steps of the spectral analysis method are described as follows:
Step 1 The Fourier coefficients for ΔN time series are determined and they are used for computing periodogram values (see Eq. 6) presented in the logarithmic scale, as shown in Fig. 4. The obtained results reveal that the totally dominant period in the investigated ΔN time series is 12 months (1 year).
Step 2 The seasonal components S F of ΔN time series are determined on the basis of Eq. (4) and Fourier coefficient obtained for the 12 month period in Step 1.
Step 3 The seasonal components are subtracted from ΔN time series. Two regression models, (a) a linear model and (b) a third degree polynomial, are then fitted to the obtained residuals, giving trend components T F of ΔN time series.
Step 4 The remaining parts of ΔN time series are regarded as unmodelled components ε.
The temporal geoid height variations and their components obtained with the use of the spectral analysis method are illustrated in Fig. 5.
According to the seasonal decomposition method, using the additive approach described in Eq. (8), ∆N time series can be presented in the following form:
where S D i is a seasonal component, T D i is a trend-cycle component, and E i presents an unmodelled component which corresponds to E t in Eq. (7).
In the seasonal decomposition method, a period of a seasonal component of the investigated time series should be known. One year periodicity in ∆N time series was found as a dominant one using the Fourier analysis. The implementation steps of the seasonal decomposition method are described as
Step 1 The ΔN time series are smoothed using a moving average with 12 month window size providing the smoothed temporal geoid height variations ΔN S.
Step 2 The ΔN S values, computed in Step 1, are subtracted from the original ΔN time series.
Step 3 The seasonal component S D is computed on the basis of the time series obtained in Step 2, i.e., ΔN − ΔN S. S D is assumed to be constant from year to year. Thus, only one value for the month is needed. The values of ΔN − ΔN S, obtained in Step 2, for a given month are averaged. Therefore, for example, S D for January is the average of all ΔN − ΔN S values for January.
Step 4 New time series obtained by subtracting the seasonal component S D from ΔN time series were smoothed using a moving average with 5 month window size and weights 1, 2, 3, 2, and 1. The output results of this step provide the long term/trend component T D of the ΔN time series.
Step 5 Finally, the remaining geoid height variation signal is regarded as an unmodelled component E computed by subtracting the obtained seasonal S D and trend-cycle T D components from the original data, i.e., ΔN time series.
The temporal geoid height variations and their components obtained with the use of the seasonal decomposition method are depicted in Fig. 6.
The results presented in Figs. 5 and 6 confirm findings from Fig. 4 indicating that dominant parts of ΔN are seasonal components. The amplitudes of these seasonal components range from 3.5 to 6 mm depending on the subarea and the method applied. They show that long term/trend components ascend in the period between January 2004 and December 2006, then descend from January 2007 to January 2009, and then ascend again in the period between February 2009 and December 2010. This indicates that the long term/trend component of ΔN for the investigated area and time period does not change linearly. The estimated goodness-of-fit in terms of R 2 (the squared Pearson correlation coefficient; e.g., Marques de Sá 2007) ranges from 53 to 61% for the third degree polynomial model, while for the linear model, it is much smaller ranging from 20 to 27% (Fig. 5). The trend values for some periods, e.g., from January 2009 to December 2010, reach up to 2 mm/year.
Modelling temporal geoid height variations
On the basis of seasonal components and trend components determined with the use of the spectral analysis method (cf. “Analysis of temporal geoid height variations”), two temporal geoid height variations models, i.e., model A and model B, were developed for each of four investigated subareas as well as for N (avg) i . These models were obtained as a summation of the seasonal and trend components of ΔN time series, a linear trend for model A, and a third degree polynomial trend for model B. The seasonal and long term/trend components of ΔN obtained using the seasonal decomposition method (cf. “Analysis of temporal geoid height variations”) were combined to develop model C. Models A, B, and C were then compared with the respective ΔN time series specified in “Computation of temporal geoid height variations”. The differences δΔN between ΔN time series and their corresponding values obtained from models A, B, and C were computed. They are shown in Fig. 7. The statistics of δΔN are given in Table 2. The correlations between ΔN time series and models A, B, and C, expressed in percent, are given in Table 3.
The results presented in Tables 2 and 3 as well as in Fig. 7 indicate that the linear function is insufficient to model trend of temporal geoid height variations. Moreover, they reveal the superiority of the seasonal decomposition method (model C) over the spectral analysis method (models A and B) for modelling temporal geoid height variations. The dispersion and the standard deviations of differences δΔN are larger by factors 2.5 and 2, respectively, when using model A than when using model C. Table 3 indicates a substantial increase in correlations between ΔN time series and models when using consecutive A, B, and C models. The correlation above 95% when using C model proves high quality modelling of temporal geoid height variations with the seasonal decomposition method. The models developed with the use of the seasonal decomposition method match substantially better to ΔN time series than models A and B based on the spectral analysis method.
Prediction of temporal geoid height variations
An empirical approach based on the result obtained from the seasonal decomposition method was implemented in this study for predicting geoid height variations. The predicted geoid height variation values ΔN (pre) were determined as the sum of seasonal component values and values of mathematical model fitted to the long term/trend component. Several mathematical models, e.g., exponential models, Fourier series, Gaussian models, sum of sines models, polynomial models, were investigated using the Matlab cftool (cf. Matlab 2015). The third degree polynomial model was chosen as a suitable one for investigated data (the estimated goodness-of-fit in terms of R 2 values is at the level of 85 to 86%) fitted to the long term/trend component. The seasonal components as well as long term/trend components were determined using ΔN time series for 6 years proceeding the prediction period.
Figure 8 shows an example of ΔN modelled using the seasonal decomposition method and predicted ΔN (pre) as well as their seasonal and long term/trend components. In this example, ΔN (avg) time series obtained in “Computation of temporal geoid height variations” for the period from January 2004 to December 2009 were used to compute ΔN (pre) for the period from January 2010 to June 2010. To obtain ΔN (pre) in different periods of the year, the procedure was repeated 12 times shifting the beginning of the time series by 1 month. Each time ΔN (pre) were predicted for the next 6 months. The differences δP between predicted geoid height variations ΔN (pre) and ΔN time series were obtained:
Figure 9 depicts ΔN time series and ΔN (pre) for 12 time series investigated. The statistics of prediction errors for all investigated cases are given in Table 4.
The results presented in Table 4 and Figs. 8 and 9 indicate that differences between prediction and data are uneven for all investigated cases. For example, when predicting geoid height variation values for 6 months from January 2010 to June 2010 using ΔN time series from January 2004 to December 2009, δP for the first 3 months ranges from −2.2 to 0.8 mm (Fig. 9a). In the other case shown in Fig. 9c, when predicting geoid height variations values for the period from March 2010 to August 2010 using ΔN time series from March 2004 to February 2010, for the first 3 months, δP ranges from −0.5 to 0.4 mm. This may indicate that differences between prediction and data strongly depend on the magnitude and the character of geoid height variations within the predicted period. The statistics presented in Table 4 reveal that the accuracy of predicted geoid height variations is about 1 mm in terms of the standard deviations of δP. Moreover, they indicate that δP is at the same level for the six subsequent months of the prediction, although its growing with time could be expected. Currently, there is a delay of 13 months in delivering monthly GRACE-based GGMs. The latest GFZ RL05 GRACE-based GGM is available for March 2016. This indicates the need for the prediction of temporal geoid height variations as well the estimation of its quality.
Summary and conclusions
The investigation of temporal variations of geoid heights, obtained from GFZ RL05 GRACE-based GGMs filtered using DDK3 filter and truncated at d/o 60, over the area of Poland divided into four 3° × 5° subareas reveals that in the absolute sense, differences between geoid height variations from epoch to epoch can reach 10 mm. In the relative sense, i.e., from one subarea to another, temporal geoid height variation differences between two neighbouring subareas can reach 2 mm at the same epoch and 11 mm at different epochs.
The obtained temporal geoid height variations for the period between January 2004 and December 2010 have been analyzed and modelled using the spectral analysis method and the seasonal decomposition method. The performed analysis indicates that the dominant period of temporal geoid height variations is 1 year with maximum values observed at the beginning of spring and minimum values at the end of summer. The linear model is not recommended to present the trend component of ∆N time series investigated. The third degree polynomial model gives better results for the investigated data. The standard deviation of unmodelled components obtained using the seasonal decomposition method is 0.5 mm, which is smaller by a factor ranging from 1.4 to 2.2 compared to the corresponding ones obtained when using the spectral analysis method. Furthermore, temporal geoid height variation models developed with the use of the results obtained using the seasonal decomposition method are considerably correlated (i.e., 95.8 to 97.2%) with geoid height variations computed using monthly GGMs. Thus, considering these results and the investigated period, the seasonal decomposition method could merely be recommended for the analysis and modelling temporal geoid height variations over the area of Poland.
The possibility of reliable prediction of geoid height variations over the area of Poland has been investigated on the basis of results obtained with the use of the seasonal decomposition method. The prediction results reveal that temporal geoid height variations at 1 mm accuracy level, in terms of the standard deviation of the differences between the predicted and given values, can be obtained for a few months (e.g., 6 months). Unusual change of the seasonal and long term/trend components of temporal geoid height variations in the predicted period may, however, lead to a large (2.3 mm in the investigated case) discrepancy between predicted temporal geoid height variations and ΔN time series data.
Temporal variations of geoid heights over the area of Poland obtained from GRACE mission data should be considered when determining a kinematic geoid model of 1 cm accuracy over this area fulfilling the needs of contemporary scientific purposes as well as high precision applications.
References
Bettadpur S (2012) UTCSR Level-2 processing standards document for level-2 product release 0005, GRACE 327–742, CSR Publ. GR-12-xx, Rev. 4.0, University of Texas at Austin
Chambers DP (2006) Evaluation of new GRACE time-variable gravity data over the ocean. Geophys Res Lett 33:17. doi:10.1029/2006GL027296
Dahle C, Flechtner F, Gruber C, König D, König R, Michalak G, Neumayer KH (2014) GFZ RL05: an improved time-series of monthly GRACE gravity field solutions, observation of the system earth from space–CHAMP, GRACE, GOCE and future missions. Adv Technol Earth Sci. doi:10.1007/978-3-642-32135-1_4
Döll P, Kaspar F, Lehner B (2003) A global hydrological model for deriving water availability indicators: model tuning and validation. J Hydrol 270(1–2):105–134
Drewes H, Kuglitsch F, Adám J, Rózsa S (2016) The Geodesist’s handbook 2016. J Geod 90(10):907–1205. doi:10.1007/s00190-016-0948-z
Flechtner F, Neumayer K-H, Dahle C, Dobslaw H, Fagiolini E, Raimondo J-C, Güntner A (2016) What can be expected from the GRACE-FO laser ranging interferometer for earth science applications? Surv Geophys 37:453–470. doi:10.1007/s10712-015-9338-y
Floberghagen R, Fehringer M, Lamarre D, Muzi D, Frommknecht B, Steiger CH, Pineiro J, da Costa A (2011) Mission design, operation and exploitation of the gravity field and steady-state ocean circulation explorer mission. J Geod 85(11):749–758. doi:10.1007/s00190-011-0498-3
Guo J, Mu D, Liu X, Yan H, Sun Z, Guo B (2016) Water storage changes over the Tibetan plateau revealed by GRACE mission. Acta Geophys 64(2):463–476. doi:10.1515/acgeo-2016-0003
Krynski J (2007) Precise quasigeoid modelling in Poland-Results and accuracy estimation (in Polish), Monographic series of the Institute of Geodesy and Cartography, Nr 13. Warsaw, Poland
Krynski J, Łyszkowicz A (2006) Centimetre quasigeoid modelling in Poland using heterogeneous data. In: Proceedings of the 1st international symposium of the international gravity field service (IGFS), August 28–September 1, 2006, in Istanbul, Turkey, 123–127
Krynski J, Kloch-Glowka G, Szelachowska M (2014) Analysis of time variations of the gravity field over Europe obtained from GRACE data in terms of geoid height and mass variations. In: Rizos C, Willis P (eds) Earth on the edge: science for a sustainable planet, IAG Symposia, vol 139. pp 365–370. doi:10.1007/978-3-642-37222-3_48
Kusche J (2007) Approximate decorrelation and non-isotropic smoothing of time variable GRACE-type gravity field models. J Geod 81(11):733–749
Kusche J, Schmidt R, Petrovic S, Rietbroek R (2009) Decorrelated GRACE time-variable gravity solutions by GFZ, and their validation using a hydrological model. J Geod 83(10):903–913
Likkason OK (2011) Spectral analysis of geophysical data. In: DongMei C (ed) Advances in data, methods, Models and their applications in geoscience, Chapt 2. InTech. ISBN: 978-953-307-737-6. doi:10.5772/28070
Luthcke SB, Sabaka TJ, Loomis BD, Arendt AA, McCarthy JJ, Camp J (2013) Antarctica, Greenland and Gulf of Alaska land-ice evolution from an iterated GRACE global mascon solution. J Glaciol 59(216):613–631. doi:10.3189/2013JoG12J147
Makridakis S, Wheelwright SC, Hyndman RJ (1998) Forecasting: methods and applications, 3rd edn. Wiley, New York. ISBN 978-0-471-53233-0
Marques de Sá JP (2007) Applied Statistics Using SPSS, STATISTICA, MATLAB and R, 2nd edn. Springer-Verlag, Berlin. doi:10.1007/978-3-540-71972-4
Matlab (2015) MATLAB version 8.6 (Matlab R2015b), Natick, The MathWorks Inc
Rangelova E (2007) A dynamic geoid model for Canada, PhD. Thesis, University of Calgary, Department of Geomatics Engineering, Report No. 20261
Rangelova E, Sideris MG (2008) Contributions of terrestrial and GRACE data to the study of the secular geoid changes in North America. J Geodyn 46(3):131–143
Rangelova E, Fotopoulos G, Sideris MG (2010) Implementing a dynamic geoid as a vertical datum for orthometric heights in Canada. In: Mertikas SPP (ed) Gravity, geoid and earth observation, IAG Commission 2 Gravity Field, Chania, Greece. IAG Symposia, 135:295–302, June 23–27, 2008, Springer
Reigber C, Lühr H, Schwintzer P (2002) CHAMP mission status. Adv Space Res 30(2):129–134. doi:10.1016/S0273-1177(02)00276-4
SESWG (2002) Living on a restless planet. NASA—Solid Earth Science Working Group (SEWSG). http://solidearth.jpl.nasa.gov/PDF/SESWG_final_combined.pdf. Accessed 15 Oct 2016
Swenson S, Wahr J (2007) Multi-sensor analysis of water storage variations in the Caspian Sea. Geophys Res Lett 34:16401. doi:10.1029/2007GL030733
Szelachowska M, Krynski J (2014) GDQM-PL13—the new gravimetric quasigeoid model for Poland. Geoinform Issues 6(1):5–19
Tapley BD, Bettadpur S, Watkins M, Reigber C (2004) The gravity recovery and climate experiment: mission overview and early results. Geophys Res Lett 31:L09607. doi:10.1029/2004GL019920
Tapley B, Flechtner F, Watkins M, Bettadpur S (2015) GRACE mission: status and prospects. The GRACE Science Team Meeting, 21–23 September 2015, Austin, Texas
Torge W, Müller J (2012) Geodesy, 4th edn. Walter de Gruyter, Berlin-Boston
Tscherning CC, Arabelos D, Strykowski G (2000) The 1-cm geoid after GOCE. In: Sideris MG (ed), Gravity, geoid and geodynamics 2000. IAG Symposia, 123, pp 267–270
Wahr J, Molenaar M, Bryan F (1998) Time variability of the Earth’s gravity field: Hydrological and oceanic effects and their possible detection using GRACE. J Geophys Res 103(B12):30205–30229
Watkins MM, Yuan D-N (2014) GRACE: JPL level-2 processing standards document for level-2 product release 05.1. GRACE 327–744 (v5.1), Jet Propulsion Laboratory, California Institute of Technology
Wei W (2006) Time series analysis: univariate and multivariate methods, 2nd edn. Pearson Addison Wesley, New York
Wu X, Heflin MB (2015) A global assessment of accelerations in surface mass transport. Geophys Res Lett 42(16):6716–6723. doi:10.1002/2015GL064941
Acknowledgements
This work was supported by the Polish National Science Centre (NCN) within the research Grant No. 2014/13/B/ST/10/02742.
Author information
Authors and Affiliations
Corresponding author
Rights and permissions
Open Access This article is distributed under the terms of the Creative Commons Attribution 4.0 International License (http://creativecommons.org/licenses/by/4.0/), which permits unrestricted use, distribution, and reproduction in any medium, provided you give appropriate credit to the original author(s) and the source, provide a link to the Creative Commons license, and indicate if changes were made.
About this article
Cite this article
Godah, W., Szelachowska, M. & Krynski, J. On the analysis of temporal geoid height variations obtained from GRACE-based GGMs over the area of Poland. Acta Geophys. 65, 713–725 (2017). https://doi.org/10.1007/s11600-017-0064-3
Received:
Accepted:
Published:
Issue Date:
DOI: https://doi.org/10.1007/s11600-017-0064-3