Abstract
Background/Objectives:
To compare linear regression coefficients adjusted for random errors with true coefficients.
Subjects/Methods:
Three hundred and two individuals from the city of Rio de Janeiro, Brazil answered 20 non-consecutive 24-hr. Means of 20 24-hr were used as an approximation of the usual dietary intakes. It was simulated outcomes with pre-defined linear regression coefficient (β=1.0, referred as 'true coefficient') for usual coffee and soft-drink intakes as explanatory variables controlled for sex and age. Regression calibration was applied in each 1000 random combinations of j days of intake (j=2, 4 and 6), and adjusted coefficients were compared with true one.
Results:
Mean-adjusted coefficients were 1.06 to 1.03 (coffee) and 1.17 to 1.11 (soft drink). The association was not detected (95% CI included zero) in 33 to 23% (coffee) and 37 to 23% (soft drink) when using two and six collection days, respectively, compared with 20% when using observed usual intake. Frequency of consumption as covariate in the regression calibration model increased the precision of the adjusted coefficients.
Conclusions:
Adjustment for random errors de-attenuates the association but its precision depends mainly on the number of collection days and sample size.
This is a preview of subscription content, access via your institution
Access options
Subscribe to this journal
Receive 12 print issues and online access
$259.00 per year
only $21.58 per issue
Buy this article
- Purchase on Springer Link
- Instant access to full article PDF
Prices may be subject to local taxes which are calculated during checkout




Similar content being viewed by others
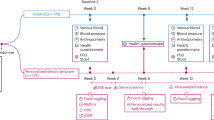
References
Willett WC . Nutritional Epidemiology. Oxford University Press: New York, 2012.
Freedman LS, Commins JM, Moler JE, Arab L, Baer DJ, Kipnis V et al. Pooled results from 5 validation studies of dietary self-report instruments using rescovery biomarkers for energy and protein intake. Am J Epidemiol 2014; 180: 172–188.
Freedman LS, Commins JM, Moler JE, Willett WC, Tinker LF, Subar AF et al. Pooled results from 5 validation studies of dietary self-report instruments using recovery biomarkers for potassium and sodium intake. Am J Epidemiol 2015; 181: 473–487.
Beaton GH, Milner J, Corey P, Feather TE, Little JA . Source of variation in 24-hour dietary recall data: implications for nutrition study design and interpretation. Am J ClinNutr 1979; 32: 2546–2459.
Dodd KW, Guenther PM, Freedman LS, Subar AF, Kipnis V, Midthue D et al. Statistical methods for estimating usual intake of nutrients and foods: a review of the theory. J Am Diet Assoc 2006; 106: 1640–1650.
Freedman LS, Schatskin A, Midthune, Kipnis V . Dealing with dietary measurement error in nutritional cohort studies. J Natl Cancer Inst 2011; 103: 1086–1092.
Tooze JA, Midthune D, Dodd KW, Krebs-Smith SM, Guenther PM, Carroll RJ et al. A new statistical method for estimating the usual intake of episodically consumed foods with application to their distribution. J Am Diet Assoc 2006; 106: 1575–1587.
Hartig U, Haubrock J, Knüppel S, Boeing H . The MSM program: web-based statistics package for estimating usual dietary intake using the Multiple Source Method. Eur J Clin Nutr 2011; 65: S87–S91.
Verly-Jr E, Oliveira DC, Fisberg RM, Marchioni DM . Performance of statistical methods to correct food intake distribution: comparison between observed and estimated usual intake. Br J Nutr 2016; 116: 897–903.
Goedhart PW, van der Voet H, Knüppel S, Dekkers ALM, Dodd KW, Boeing H et al. A comparison by simulation of different methods to estimate the usual intake distribution for episodically consumed foods. Supporting Publications 2012:EN-299 (65pp). Available at: www.efsa.europa.eu/publications.
Kipnis V, Midthune D, Buckman DW, Dodd KW, Guenther PM, Krebs-Smith SM et al. Modeling data with excess zeros and measurement error: application to evaluating relationships between episodically consumed foods and health outcomes. Biometrics 2009; 65: 1003–1010.
Conway JM, Ingwersen LA, Vinyard BT, Moshfegh AJ . Effectiveness of the US Department of Agriculture 5-step multiple-pass method in assessing food intake in obese and non obese women. Am J Clin Nutr 2003; 77: 1171–1178.
Subar AM, Dodd KW, Guenther PM, Kipnis V, Midthune D, VcDowell M et al. The food propensity questionnaire: concept, development, and validation for use as covariate in a model to estimate usual food intake. J Am Diet Assoc 2006; 106: 1556–1563.
Cohen J . Statistical Power Analysis for the Behavioral Sciences, 2nd edn. Lawrence Erlbaum Associates: Mahwah, NJ, 1988.
Carroll RJ, Midthune D, Subar AF, Shumkovich M, Freedman LS, Thompson FE et al. Taking advantages of the strengths of 2 different dietary assessment instruments to improve estimates for nutritional epidemiology. Am J Epidemiol 2012; 175: 340–347.
Kipnis V, Midthune D, Freedman L, Bigham S, Day NE, Riboli E et al. Bias in dietary-report instruments and its implications for nutritional epidemiology. Public Health Nutr 2002; 5: 915–923.
Kipnis V, Subar A, Midthune D . Structure of dietary measurement error: results of the OPEN Biomarker Study. Am J Epidemiol 2003; 158: 14–21.
Moshfegh AJ, Rhodes DG, Baer DJ, Murayi T, Clemens J, Rumpler WV et al. The US Department of Agriculture Automated Multiple-Pass Method reduces bias in the collection of energy intakes. Am J Clin Nutr 2008; 88: 324–332.
Acknowledgements
This work was funded by State of Rio de Janeiro Research Foundation (n. E-26/201.488/2014) and Brazilian National Research Council (n. 481434/2013-5).
Authors contribution
EVJ conceptualized the study question, designed the research and analyzed data; VTB and RS reviewed the analyses and the manuscript and provided comments. EVJ had primary responsibility for final content. All authors read and approved the final manuscript. Neither author reported a conflict of interest related to the study.
Author information
Authors and Affiliations
Corresponding author
Ethics declarations
Competing interests
The authors declare no conflict of interest.
Rights and permissions
About this article
Cite this article
Verly-Jr, E., Sichieri, R. & Baltar, V. Adjusting diet–outcome associations for random error: comparison of associations based on observed and estimated usual intakes. Eur J Clin Nutr 71, 1418–1422 (2017). https://doi.org/10.1038/ejcn.2017.120
Received:
Revised:
Accepted:
Published:
Issue Date:
DOI: https://doi.org/10.1038/ejcn.2017.120
This article is cited by
-
Accounting for nutrition-related health impacts in food life cycle assessment: insights from an expert workshop
The International Journal of Life Cycle Assessment (2024)