Abstract
The success of self-attention in NLP has led to recent applications in end-to-end encoder-decoder architectures for speech recognition. The transformer model based on self-attention get a promising result. As you can see, the hybrid model based on Connectionist Temporal Classification (CTC)/Attention has very prominent advantages in decoding, which can combine the excellent sequence-to-sequence modeling ability of attention, and can also combine CTC to achieve temporal alignment. We propose SA-Conv-CTC/Attention model, which apply a Self-Attention and shallow Convolution based hybrid encoder to Hybrid CTC/Attention Architecture, and we also explored the method of decoding with Self-Attention language models. The CTC network sits on top of the encoder and is jointly trained with the attention-based decoder, even participated in decoding. We achieve a 0.8-4.75% error reduction compared to other hybrid CTC/Attention systems on WSJ and HKUST dataset.
Export citation and abstract BibTeX RIS
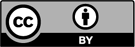
Content from this work may be used under the terms of the Creative Commons Attribution 3.0 licence. Any further distribution of this work must maintain attribution to the author(s) and the title of the work, journal citation and DOI.