Abstract
Classification of stars is essential to investigate the characteristics and behavior of stars. Performing classifications manually is error-prone and time-consuming. Machine learning provides a computerized solution to handle huge volumes of data with minimal human input. k-Nearest Neighbor (kNN) is one of the simplest supervised learning approaches in machine learning. This paper aims at studying and analyzing the performance of the kNN algorithm on the star dataset. In this paper, we have analyzed the accuracy of the kNN algorithm by considering various distance metrics and the range of k values. Minkowski, Euclidean, Manhattan, Chebyshev, Cosine, Jaccard, and Hamming distance were applied on kNN classifiers for different k values. It is observed that Cosine distance works better than the other distance metrics on star categorization.
Export citation and abstract BibTeX RIS
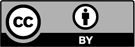
Content from this work may be used under the terms of the Creative Commons Attribution 3.0 licence. Any further distribution of this work must maintain attribution to the author(s) and the title of the work, journal citation and DOI.