Development of environmental economy and management in the age of AI based on green finance
- Russian State Agrarian University—Moscow Timiryazev Agricultural Academy (RSAU—MAA named after K. A. Timiryazev), Moscow, Russia
Introduction
Green finance is a set of innovative solutions in the financial sector of the economy, which have become widespread due to the adoption by the United Nations (UN) of the Sustainable Development Goals (SDGs) in 2015, which is expected to reach its peak in the Decade of Action (Tong A et al., 2022; Zhou and Tang, 2022). Green finance refers to the totality of financial transactions (including public subsidies, private investment, lending, charitable contributions, and payment for goods and services by consumers) that comply with ESG principles and are carried out with corporate environmental responsibility by all subjects of financial relations (Dong et al., 2022; Ronaldo and Suryanto, 2022; Tang M et al., 2022; Wang K et al., 2022).
There are currently all necessary institutional conditions and prerequisites for the development of green finance. Governments worldwide have ratified the SDGs and adopted national strategies to decarbonize their economies (Feng et al., 2022; Li J et al., 2022). Businesses actively publish corporate sustainability and environmental responsibility reports, following ESG principles in their operations and building green business practices to their competitive advantage (Jiang et al., 2022; Li S et al., 2022). In civil society and consumer society, environmentally responsible communities are being created and developed; entire areas (cities and regions) become environmentally sustainable, for example, by practising recycling and clean energy (Desalegn and Tangl, 2022; Guo H et al., 2022; Popkova, 2022; Sun and Chen, 2022; Wu G, 2022).
Nevertheless, green finance continues to have a limited impact on the environmental economy and management (Yang and Masron, 2022; Zhang and Liu, 2022). The causal relationships between the spread and use of green finance remain largely undefined, which is a gap in the literature. This raises the research question (RQ) of what importance green finance has for the economy and environmental management and the prospects for increasing this importance.
In seeking an answer to the posed RQ, this research considers the special context of the Decade of Action because it takes place in the era of the economy of artificial intelligence (AI). The essence of the AI economy consists in the automatization of economic processes and dissemination of smart technologies, which are based on AI. As such, green finance is undergoing a digital upgrade, in which it is becoming increasingly smart. Consideration of the context of the AI era ensures the originality of this research. This research aims to explore the importance of green finance in the AI era for economics and environmental management.
Literature review
The theoretical basis of this research is the concept of green finance. The scientific provisions of this concept are detailed and described in detail in existing publications (Guang-Wen and Siddik, 2022; Guo C Q et al., 2022; Kaginalkar et al., 2022; Li Q et al., 2022; Ma et al., 2022; Wu H, 2022), which allows us to determine the degree of elaboration of the problem of this research as a high. The conducted literature review and content analysis of existing works on the given topic showed that the existing literature notes a fragmented contribution of green finance to the economy and environmental management.
We understand the environmental economy as a sphere of the economy in which green innovations are actively implemented and sustainable economic growth is achieved. Environmental management is a process of business management with the implementation of environmentally responsible organisational models and circular business practices. Environmental economy and management facilitate the achievement of the Sustainable Development Goals (SDGs), which are connected with environment protection (development of clean energy, protection of ecosystems, fight against climate change, etc.) (Huang et al., 2022; Li X et al., 2022; Li Z et al., 2022; Liu and Tang, 2022; Pang et al., 2022; Tong J et al., 2022; Zhang et al., 2022).
Chang et al. (2022), Chen et al. (2022), Tang (2022), and Wang Y et al. (2022) note that the contribution of green finance to the development of the economy and environmental management is achieved through its particularity of supporting ESG principles and the dissemination of corporate environmental responsibility. However, the existing literature leaves the RQ open and does not sufficiently reveal the specifics of green finance development in the AI era. The lack of elaboration on the causal relationships between the development of green finance in the AI era and its importance to economics and environmental management is a gap in the literature that this research seeks to fill.
Based on the works of Chen and Zhou (2021), Chen (2021), and Natanelov et al. (2022), which noted the benefits of smart finance, the research hypothesizes that the development of green finance in the AI era is constrained by the lack of use of advanced smart technology capabilities; the prospects for the development of green finance are related to the increased use of these capabilities. To fill the gap, this research systemically examines the implications of green finance for the entire set of SDGs shaping the green economy and management and considers the advantages of smart green finance as a new kind of finance that has emerged in the AI era.
Materials and methodology
To solve the first research problem, the authors model the contribution of green finance to economic development and environmental management using the structural equation modelling method (SEM). For this purpose, using the methods of correlation and regression analysis, the authors determine the impact of green finance factors allocated to the Green Finance Platform, (2021) [“policy and strategy” (ps), “market and product” (mp), and “international cooperation” (ic)] in 2021 on the results of environmental economy and management from a sustainable development perspective, i.e., scores of SDGs 2–3, 6–7, and 11–15 (UNDP, 2021) in 2022.
To solve the second research problem, the authors studied the international case studies of the development of smart green finance in the AI era. The sources of information, evidence, and case studies are IDOM (2020), Vinogradova (2021), and Damianova et al. (2018). This allows specifying the role of smart technologies in the development of green finance in the age of AI and identifying the consequences of their development for traditional finance.
Results
Modelling the contribution of green finance to economic development and environmental management
In the first task of this research, the authors performed economic and mathematical modelling of the contribution of green finance to the development of the economy and environmental management (Supplementary Material).As a result, nine multiple linear regression equations were obtained, each of which was tested for reliability using Fisher’s F-test. Detailedregression statistics are given along with the Data Table in the Microsoft Excel file that accompanies this research. To systemically reflect and comprehensively demonstrate the impact of green finance on the economy and environmental management in 2022, the authors present the results using the SEM model in Figure 1, with correlation coefficients in italics and regression coefficients in regular font.
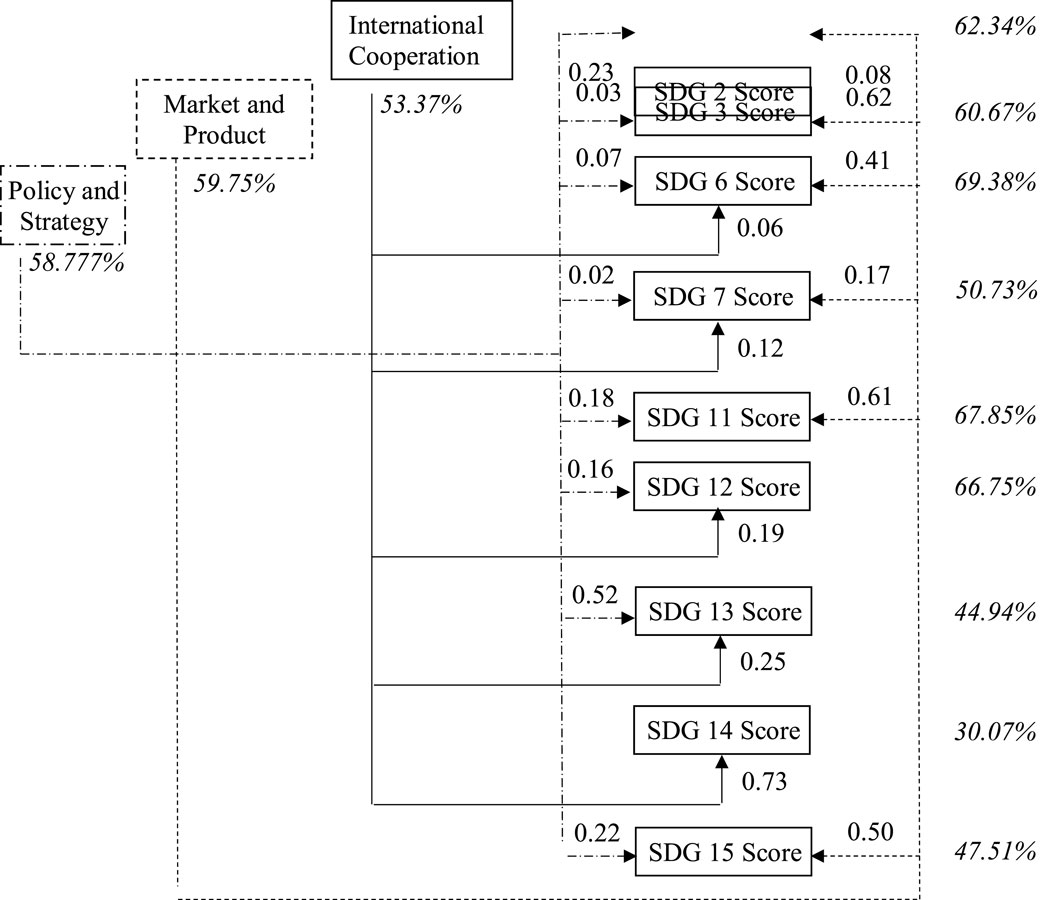
FIGURE 1. SEM model of the impact of green finance on the economy and environmental managementin 2022.
The SEM model in Figure 1 reflects the following results: the SDG 2 Score = 73.91–0.23*ps-0.08*mp+0.01*ic. According to this equation, the SDG 2 Score increases by 0.23 points when policy and strategy increase by 1. The SDG 2 Score increases by 0.08 points if the market and product increase by 1. Correlation is high: the SDG 2 Score is influenced by green finance factors by 62.34%. The significance is F = 1.7*10–0.5; therefore, the equation corresponds to the highest level of significance 0.01. At a given significance level with three factor variables (k1 = 3) and 54 observations (k2 = 54-3-1 = 50), the tabulated F is 4.126. The observed F is 10.59, exceeding the tabulated F. In this regard, the F-test is passed, and the equation is reliable.
The SDG 3 Score = 96.06–0.03*ps-0.62*mp+0.15*ic. According to this equation, the SDG 3 Score increases by 0.03 points when the policy and strategy increase by 1. The SDG 3 Score increases by 0.62 points if the market and product increase by 1. Correlation is high: the SDG 3 Score is influenced by green finance factors by 60.67%. The significance is F = 3.7*10–0.5; therefore, the equation corresponds to the highest level of significance 0.01. At a given significance level with three factor variables (k1 = 3) and 54 observations (k2 = 54-3-1 = 50), the tabulated F is 4.126. The observed F is 9.71, exceeding the tabulated F. In this regard, the F-test is passed, and the equation is reliable.
The SDG 3 Score = 92.71–0.07*ps-0.41*mp-0.06*ic. According to this equation, the SDG 3 Score increases by 0.07 points when the policy and strategy increase by 1. The SDG 6 Score increases by 0.41 points if the market and product increase by 1. The SDG 6 Score increases by 0.06 points if international cooperation increases by 1. Correlation is high: the SDG six Score is influenced by green finance factors by 69.37%. The significance is F = 3*10–0.7; therefore, the equation corresponds to the highest level of significance 0.01; therefore, the equation corresponds to the highest level of significance 0.01. At a given significance level with three factor variables (k1 = 3) and 54 observations (k2 = 54-3-1 = 50), the tabulated F is 4.126. The observed F is 15.46, exceeding the tabulated F. In this regard, the F-test is passed, and the equation is reliable.
The SDG 7 Score = 81.85–0.02*ps-0.17*mp-0.12*ic. According to this equation, the SDG 7 Score increases by 0.02 points when the policy and strategy increase by 1. The SDG 7 Score increases by 0.17 points if the market and product increase by 1. The SDG 7 Score increases by 0.12 points if international cooperation increases by 1. Correlation is moderate: the SDG 7 Score is influenced by green finance factors by 50.73%. The significance is F = 0.002; therefore, the equation corresponds to the highest level of significance 0.01. At a given significance level with three factor variables (k1 = 3) and 54 observations (k2 = 54-3-1 = 50), the tabulated F is 4.126. The observed F is 5.77, exceeding the tabulated F. In this regard, the F-test is passed, and the equation is reliable.
The SDG 11 Score = 97.28–0.18*ps-0.61*mp+0.18*ic. According to this equation, the SDG 11 Score increases by 0.18 points when the policy and strategy increase by 1. The SDG 11 Score increases by 0.61 points if the market and product increase by 1. Correlation is high: the SDG 11 Score is influenced by green finance factors by 67.85%. The significance is F = 8*10–0.7; therefore, the equation corresponds to the highest level of significance 0.01. At a given significance level with three factor variables (k1 = 3) and 54 observations (k2 = 54-3-1 = 50), the tabulated F is 4.126. The observed F is 14.22, exceeding the tabulated F. In this regard, the F-test is passed, and the equation is reliable.
The SDG 12 Score = 63.18–0.16*ps+0.84*mp-0.19*ic. According to this equation, the SDG 12 Score increases by 0.16 points when the policy and strategy increase by 1. The SDG 12 Score increases by 0.19 points if the market and product increase by 1. Correlation is high: the SDG 12 Score is influenced by green finance factors by 66.75%. The significance is F = 1.5*10–0.6; therefore, the equation corresponds to the highest level of significance 0.01. At a given significance level with three factor variables (k1 = 3) and 54 observations (k2 = 54-3-1 = 50), the tabulated F is 4.126. The observed F is 13.40, exceeding the tabulated F. In this regard, the F-test is passed, and the equation is reliable. The SDG 13 Score = 63.17–0.52*ps+1.04*mp-0.25*ic. According to this equation, the SDG 13 Score increases by 0.52 points when the policy and strategy increase by 1. The SDG 13 Score increases by 0.25 points if the market and product increase by 1. Correlation is moderate: the SDG 13 Score is influenced by green finance factors by 66.75%. The significance is F = 0.009; therefore, the equation corresponds to the highest level of significance 0.01. At a given significance level with three factor variables (k1 = 3) and 54 observations (k2 = 54-3-1 = 50), the tabulated F is 4.126. The observed F is 14.22, exceeding the tabulated F. In this regard, the F-test is passed, and the equationis reliable.
The SDG 14 Score = 55.75 + 0.34*ps+0.43*mp-0.73*ic. According to this equation, the SDG 14 Score increases by 0.73 points when the policy and strategy increase by 1. Correlation is moderate: The SDG 14 Score is influenced by green finance factors by 35.06%. The significance is F = 0.08; therefore, the equation corresponds to the highest level of significance 0.01. At a given significance level with three factor variables (k1 = 3) and 54 observations (k2 = 54-3-1 = 50), the tabulated F is 2.23. The observed F is 2.34, exceeding the tabulated F. In this regard, the F-test is passed, and the equation is reliable.
The SDG 15 Score = 73.13–0.22*ps-0.50*mp+0.43*ic. According to this equation, the SDG 15 Score increases by 0.22 points when the policy and strategy increase by 1. The SDG 15 Score increases by 0.50 points if the market and product increase by 1. Correlation is moderate: The SDG 14 Score is influenced by green finance factors by 47.51%. The significance is F = 0.005; therefore, the equation corresponds to the highest level of significance 0.01. At a given significance level with three factor variables (k1 = 3) and 54 observations (k2 = 54-3-1 = 50), the tabulated F is 4.126. The observed F is 4.85, exceeding the tabulated F. In this regard, the F-test is passed, and the equation is reliable.
The cumulative contribution of each factor of green finance (separately) to the development of ecological economy and management is defined as follows:
The results indicate that green finance significantly contributes to the development of the economy and environmental management, supporting the implementation of the entire set of environmental SDGs. The discovered systemic contribution of green finance to the achievement of environmental Sustainable Development Goals allows for a wider (than before) use of green finance in the interests of the development of the economy and management of the environment.
Case study of smart green finance development in the AI era
In the second task of this research, let us look at international case studies of the development of smart green finance in the AI era. In Russia, green finance is actively used to develop alternative energy and waste recycling. ROSATOM has been issuing green bonds since 2021 as an ESG tool for green financing of wind energy facilities. In the North of Russia, in the Republic of Yakutia, a comprehensive program for the development of solar energy based on green financing is being implemented. The Yakutsk solar power plant was built based on a green loan provided by “Otkritie” Bank to the “Enelt” Group for the implementation of the project of “RusHydro” Corporation. Both projects represent the Smart Grid. In the Yegoryevsk district (Moscow Region), thanks to green investments, the group of companies “Ecoline” will build the largest in Eastern Europe automated waste recycling complex on the territory of ecotechnopark “Vostok” in 2019–2020 (Vinogradova, 2021).
In 2014, China created a green finance task force to support the decarbonization of the economy. First, information systems and big data, which store information about credit histories in the field of green finance, play an essential role in this process. Second, an important role is played by smart green finance (Damianova et al., 2018). According to case studies, the development of the environmental economy and management in the AI era must be based on smart green finance.
Discussion
The research contributes to the literature by developing scientific provisions of the concept of green finance, clarifying the causal relationships of their distribution and use based on international experience. In contrast to Huang et al. (2022), Li Z et al. (2022), Liu and Tang (2022), Pang et al. (2022), Tong J et al. (2022), and Zhang et al. (2022), this research justifies that green finance makes a holistic and integrated contribution to economic and environmental management, rather than a stand-alone contribution.
In contrast to Chang et al. (2022), Chen et al. (2022), Tang (2022), and Wang Y et al. (2022), it has been proven that the spread of green finance is significantly affected by its accessibility, which is enhanced by the use of advanced automation tools. This means that the importance of green finance for the economy and environmental management in the AI era is determined not by institutions (ESG principles and attractiveness to market actors) but by technology. Due to its high-tech nature, smart green finance has become massively available and could supplant traditional finance.
Conclusion
The main contribution of this paper to the literature is the discovery and description of a complex chain of cause-and-effect relationships of the development of green finance in the age of AI. The case study of the international experience of developed and developing countries substantiates that the development of green finance in the AI era is constrained by insufficient use of advanced capabilities of smart technologies. The prospects for the development of smart technologies are associated with the expansion of the use of these capabilities.
The scientific novelty and originality of the research results lie in the fact that the composed econometric model SEM, for the first time, reflected the systemic relationship of green finance with the economy and environmental management through the prism of the set of environmental SDGs. The theoretical significance of the research is that it reveals the peculiarity of green finance in the AI era, namely that green finance can reach its full development potential only by relying on smart technology.
The practical significance of the research lies in the fact that the conclusion made about the increased use of smart technologies will increase the competitiveness of green finance and accelerate its spread in the economy and environmental management. The case studies discussed will be useful for other countries in the world. The results of this research will contribute to the ongoing discussions in the sphere of environmental science on the topic of environmental economics and management by a discovery of a new tool of the management of environmental economy and business. This tool is green finance.
Author contributions
All authors listed have made a substantial, direct, and intellectual contribution to the work and approved it for publication.
Conflict of interest
The authors declare that the research was conducted in the absence of any commercial or financial relationships that could be construed as a potential conflict of interest.
Publisher’s note
All claims expressed in this article are solely those of the authors and do not necessarily represent those of their affiliated organizations, or those of the publisher, the editors and the reviewers. Any product that may be evaluated in this article, or claim that may be made by its manufacturer, is not guaranteed or endorsed by the publisher.
References
Chang, X., Fu, K., Jin, Y., and Liem, P. F. (2022). Sustainable finance: ESG/CSR, firm value, and investment returns. Asia-Pacific J. Financial Stud. 51 (3), 325–371. doi:10.1111/ajfs.12379
Chen, C., and Zhou, Y. (2021). Application of deep reinforcement learning algorithm in smart finance. Front. Artif. Intell. Appl. 341, 40–48. doi:10.3233/FAIA210230
Chen, Y. (2021). Framework of the smart finance and accounting management model under the artificial intelligence perspective. Mob. Inf. Syst. 2021 (SI), 4295191–4295211. doi:10.1155/2021/4295191
Chen, Y. P. V., Zhuo, Z., Huang, Z., and Li, W. (2022). Environmental regulation and ESG of SMEs in China: Porter hypothesis re-tested. Sci. Total Environ. 850, 157967. doi:10.1016/j.scitotenv.2022.157967
Damianova, A., Guttierez, E., Levitanskaya, K., Minasyan, G., and Nemova, V. (2018). Russia green finance: Unlocking opportunities for green investments. Moscow, Russia: World Bank Group. Available at: https://documents1.worldbank.org/curated/en/699051540925687477/pdf/131516-RUSSIAN-PN-P168296-P164837-PUBLIC-Green-finance-Note.pdf (Accessed October 22, 2022).
Desalegn, G., and Tangl, A. (2022). Enhancing green finance for inclusive green growth: A systematic approach. Sustainability 14 (12), 7416. doi:10.3390/su14127416
Dong, Z., Xu, H., Zhang, Z., Lyu, Y., Lu, Y., and Duan, H. (2022). Whether green finance improves green innovation of listed companies—evidence from China. Int. J. Environ. Res. Public Health 19 (17), 10882. doi:10.3390/ijerph191710882
Feng, H., Liu, Z., Wu, J., Iqbal, W., Ahmad, W., and Marie, M. (2022). Nexus between Government spending’s and Green Economic performance: Role of green finance and structure effect. Environ. Technol. Innovation 27, 102461. doi:10.1016/j.eti.2022.102461
Green Finance Platform (2021). Global finance and development report. Available at: https://www.greenfinanceplatform.org/research/global-finance-and-development-report-2021 (Accessed October 22, 2022).
Guang-Wen, Z., and Siddik, A. B. (2022). Do corporate social responsibility practices and green finance dimensions determine environmental performance? An empirical study on Bangladeshi banking institutions. Front. Environ. Sci. 10, 890096. doi:10.3389/fenvs.2022.890096
Guo, C. -Q., Wang, X., Cao, D. -D., and Hou, Y. -G. (2022). The impact of green finance on carbon emission-analysis based on mediation effect and spatial effect. Front. Environ. Sci. 10, 844988. doi:10.3389/fenvs.2022.844988
Guo, H., Gu, F., Peng, Y., Deng, X., and Guo, L. (2022). Does digital inclusive finance effectively promote agricultural green development? – a case study of China. Int. J. Environ. Res. Public Health 19 (12), 6982. doi:10.3390/ijerph19126982
Huang, H., Mbanyele, W., Wang, F., Song, M., and Wang, Y. (2022). Climbing the quality ladder of green innovation: Does green finance matter? Technol. Forecast. Soc. Change 184, 122007. doi:10.1016/j.techfore.2022.122007
Jiang, Y., Guo, C., and Wu, Y. (2022). Does digital finance improve the green investment of Chinese listed heavily polluting companies? The perspective of corporate financialization. Environ. Sci. Pollut. Res. 29 (47), 71047–71063. doi:10.1007/s11356-022-20803-z
Kaginalkar, A., Kumar, S., Gargava, P., Kharkar, N., and Niyogi, D. (2022). SmartAirQ: A big data governance framework for urban air quality management in smart cities. Front. Environ. Sci. 10, 785129. doi:10.3389/fenvs.2022.785129
Li, J., Dong, X., and Dong, K. (2022). How much does financial inclusion contribute to renewable energy growth? Ways to realize green finance in China. Renew. Energy 198, 760–771. doi:10.1016/j.renene.2022.08.097
Li, Q., Sharif, A., Razzaq, A., and Yu, Y. (2022). Do climate technology, financialization, and sustainable finance impede environmental challenges? Evidence from G10 economies. Technol. Forecast. Soc. Change 185, 122095. doi:10.1016/j.techfore.2022.122095
Li, S., Yu, Y., Jahanger, A., Usman, M., and Ning, Y. (2022). The impact of green investment, technological innovation, and globalization on CO2 emissions: Evidence from MINT countries. Front. Environ. Sci. 10, 868704. doi:10.3389/fenvs.2022.868704
Li, X., Shao, X., Chang, T., and Albu, L. L. (2022). Does digital finance promote the green innovation of China’s listed companies? Energy Econ. 114, 106254. doi:10.1016/j.eneco.2022.106254
Li, Z., Wu, L., Zhang, Z., Chen, R., Jiang, Y., Peng, Y., et al. (2022). The transformative impacts of green finance governance on construction-related CO2 emissions. Sustainability 14 (16), 9853. doi:10.3390/su14169853
Liu, Q., and Tang, L. (2022). Research on the accelerating effect of green finance on the transformation of energy consumption in China. Res. Int. Bus. Finance 63, 101771. doi:10.1016/j.ribaf.2022.101771
Ma, R., Li, F., and Du, M. (2022). How does environmental regulation and digital finance affect green technological innovation: Evidence from China. Front. Environ. Sci. 10, 928320. doi:10.3389/fenvs.2022.928320
Natanelov, V., Cao, S., Foth, M., and Dulleck, U. (2022). Blockchain smart contracts for supply chain finance: Mapping the innovation potential in Australia-China beef supply chains. J. Industrial Inf. Integration 30, 100389. doi:10.1016/j.jii.2022.100389
Pang, L., Zhu, M. N., and Yu, H. (2022). Is green finance really a blessing for green technology and carbon efficiency? Energy Econ. 114, 106272. doi:10.1016/j.eneco.2022.106272
Popkova, E. (2022). Advanced issues in the green economy and Sustainable development in emerging market economies (elements in the economics of emerging markets). Cambridge, UK: Cambridge University Press. doi:10.1017/9781009093408
Ronaldo, R., and Suryanto, T. (2022). Green finance and sustainability development goals in Indonesian Fund Village. Resour. Policy 78, 102839. doi:10.1016/j.resourpol.2022.102839
Sun, H., and Chen, F. (2022). The impact of green finance on China’s regional energy consumption structure based on system GMM. Resour. Policy 76, 102588. doi:10.1016/j.resourpol.2022.102588
Tang, H. (2022). The effect of ESG performance on corporate innovation in China: The mediating role of financial constraints and agency cost. Sustainability 14 (7), 3769. doi:10.3390/su14073769
Tang, M., Ding, J., Kong, H., Bethel, B. J., and Tang, D. (2022). Influence of green finance on ecological environment quality in Yangtze River Delta. Int. J. Environ. Res. Public Health 19 (17), 10692. doi:10.3390/ijerph191710692
Tong, A., Jiang, L., Ru, Y., Hu, Z., Xu, Z., and Wang, Y. (2022). Research on the impact of inclusive finance on agricultural green development: Empirical analysis of China’s main grain producing areas. PLoS ONE 17 (9), e0274453. doi:10.1371/journal.pone.0274453
Tong, J., Yue, T., and Xue, J. (2022). Carbon taxes and a guidance-oriented green finance approach in China: Path to carbon peak. J. Clean. Prod. 367, 133050. doi:10.1016/j.jclepro.2022.133050
UNDP (2021). Sustainable development report (SDG index) 2021. Available: https://www.sdgindex.org/reports/sustainable-development-report-2021/(Accessed October 22, 2022).
Vinogradova, E. (2021). How ESG funding helps solve environmental problems. Vedomosti. Available at: https://www.vedomosti.ru/partner/articles/2021/12/10/899949-esg-finansirovanie (Accessed October 22, 2022).
Wang, K.-H., Zhao, Y.-X., Jiang, C.-F., and Li, Z.-Z. (2022). Does green finance inspire sustainable development? Evidence from a global perspective. Econ. Analysis Policy 75, 412–426. doi:10.1016/j.eap.2022.06.002
Wang, Y., Chen, L., and Jin, S. (2022). Environmental performance, social responsibility and corporate governance (ESG) ratings and financial risk. Environ. Eng. Manag. J. 21 (5), 857–868. doi:10.30638/eemj.2022.078
Wu, G. (2022). Research on the spatial impact of green finance on the ecological development of Chinese economy. Front. Environ. Sci. 10, 887896. doi:10.3389/fenvs.2022.887896
Wu, H. (2022). Trade openness, green finance and natural resources: A literature review. Resour. Policy 78, 102801. doi:10.1016/j.resourpol.2022.102801
Yang, C., and Masron, T. A. (2022). Impact of digital finance on energy efficiency in the context of green sustainable development. Sustainability 14 (18), 11250. doi:10.3390/su141811250
Zhang, D., Mohsin, M., and Taghizadeh-Hesary, F. (2022). Does green finance counteract the climate change mitigation: Asymmetric effect of renewable energy investment and R&D. Energy Econ. 113, 106183. doi:10.1016/j.eneco.2022.106183
Zhang, M., and Liu, Y. (2022). Influence of digital finance and green technology innovation on China’s carbon emission efficiency: Empirical analysis based on spatial metrology. Sci. Total Environ. 838, 156463. doi:10.1016/j.scitotenv.2022.156463
Keywords: green finance, smart technology, AI era, environmental economics, environmental management
Citation: Trukhachev VI and Dzhikiya M (2023) Development of environmental economy and management in the age of AI based on green finance. Front. Environ. Sci. 10:1087034. doi: 10.3389/fenvs.2022.1087034
Received: 01 November 2022; Accepted: 05 December 2022;
Published: 30 January 2023.
Edited by:
Bruno Sergi, Harvard University, United StatesReviewed by:
Aktam Burkhanov, Tashkent State Economic University, UzbekistanCopyright © 2023 Trukhachev and Dzhikiya. This is an open-access article distributed under the terms of the Creative Commons Attribution License (CC BY). The use, distribution or reproduction in other forums is permitted, provided the original author(s) and the copyright owner(s) are credited and that the original publication in this journal is cited, in accordance with accepted academic practice. No use, distribution or reproduction is permitted which does not comply with these terms.
*Correspondence: Meri Dzhikiya, marydzhikia@list.ru