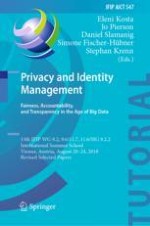
2019 | OriginalPaper | Chapter
A Causal Bayesian Networks Viewpoint on Fairness
Authors : Silvia Chiappa, William S. Isaac
Published in: Privacy and Identity Management. Fairness, Accountability, and Transparency in the Age of Big Data
Publisher: Springer International Publishing
Activate our intelligent search to find suitable subject content or patents.
Select sections of text to find matching patents with Artificial Intelligence. powered by
Select sections of text to find additional relevant content using AI-assisted search. powered by