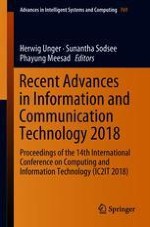
2019 | OriginalPaper | Chapter
Combining Multiple Features for Product Categorisation by Multiple Kernel Learning
Authors : Chanawee Chavaltada, Kitsuchart Pasupa, David R. Hardoon
Published in: Recent Advances in Information and Communication Technology 2018
Publisher: Springer International Publishing
Activate our intelligent search to find suitable subject content or patents.
Select sections of text to find matching patents with Artificial Intelligence. powered by
Select sections of text to find additional relevant content using AI-assisted search. powered by