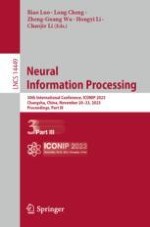
2024 | OriginalPaper | Chapter
Efficient Lightweight Network with Transformer-Based Distillation for Micro-crack Detection of Solar Cells
Authors : Xiangying Xie, Xinyue Liu, QiXiang Chen, Biao Leng
Published in: Neural Information Processing
Publisher: Springer Nature Singapore
Activate our intelligent search to find suitable subject content or patents.
Select sections of text to find matching patents with Artificial Intelligence. powered by
Select sections of text to find additional relevant content using AI-assisted search. powered by