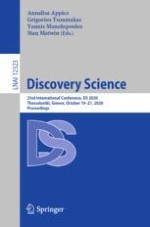
2020 | OriginalPaper | Chapter
Evaluating Decision Makers over Selectively Labelled Data: A Causal Modelling Approach
Authors : Riku Laine, Antti Hyttinen, Michael Mathioudakis
Published in: Discovery Science
Publisher: Springer International Publishing
Activate our intelligent search to find suitable subject content or patents.
Select sections of text to find matching patents with Artificial Intelligence. powered by
Select sections of text to find additional relevant content using AI-assisted search. powered by