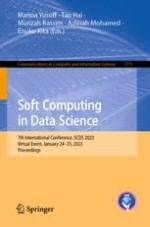
2023 | OriginalPaper | Chapter
Explainability for Clustering Models
Authors : Mahima Arora, Ankush Chopra
Published in: Soft Computing in Data Science
Publisher: Springer Nature Singapore
Activate our intelligent search to find suitable subject content or patents.
Select sections of text to find matching patents with Artificial Intelligence. powered by
Select sections of text to find additional relevant content using AI-assisted search. powered by