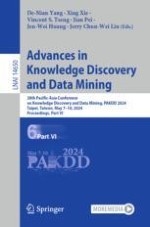
2024 | OriginalPaper | Chapter
FR\(^3\)LS: A Forecasting Model with Robust and Reduced Redundancy Latent Series
Authors : Abdallah Aaraba, Shengrui Wang, Jean-Marc Patenaude
Published in: Advances in Knowledge Discovery and Data Mining
Publisher: Springer Nature Singapore
Activate our intelligent search to find suitable subject content or patents.
Select sections of text to find matching patents with Artificial Intelligence. powered by
Select sections of text to find additional relevant content using AI-assisted search. powered by