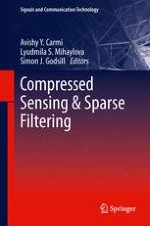
2014 | OriginalPaper | Chapter
1. Introduction to Compressed Sensing and Sparse Filtering
Authors : Avishy Y. Carmi, Lyudmila S. Mihaylova, Simon J. Godsill
Published in: Compressed Sensing & Sparse Filtering
Publisher: Springer Berlin Heidelberg
Activate our intelligent search to find suitable subject content or patents.
Select sections of text to find matching patents with Artificial Intelligence. powered by
Select sections of text to find additional relevant content using AI-assisted search. powered by