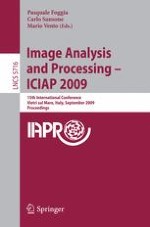
2009 | OriginalPaper | Chapter
Learning with Missing or Incomplete Data
Author : Bogdan Gabrys
Published in: Image Analysis and Processing – ICIAP 2009
Publisher: Springer Berlin Heidelberg
Activate our intelligent search to find suitable subject content or patents.
Select sections of text to find matching patents with Artificial Intelligence. powered by
Select sections of text to find additional relevant content using AI-assisted search. powered by
The problem of learning with missing or incomplete data has received a lot of attention in the literature [6,10,13,21,23]. The reasons for missing data can be multi-fold ranging from sensor failures in engineering applications to deliberate withholding of some information in medical questioners in the case of missing input feature values or lack of solved (labelled) cases required in supervised learning algorithms in the case of missing labels. And though such problems are very interesting from the practical and theoretical point of view, there are very few pattern recognition techniques which can deal with missing values in a straightforward and efficient manner. It is in a sharp contrast to the very efficient way in which humans deal with unknown data and are able to perform various pattern recognition tasks given only a subset of input features or few labelled reference cases.
In the context of pattern recognition or classification systems the problem of missing labels and the problem of missing features are very often treated separately.
The availability or otherwise of labels determines the type of the learning algorithm that can be used and has led to the well known split into supervised, unsupervised or more recently introduced hybrid/semi-supervised classes of learning algorithms.
Commonly, using supervised learning algorithms enables designing of robust and well performing classifiers. Unfortunately, in many real world applications labelling of the data is costly and thus possible only to some extent. Unlabelled data on the other hand is often available in large quantities but a classifier built using unsupervised learning is likely to demonstrate performance inferior to its supervised counterpart. The interest in a mixed supervised and unsupervised learning is thus a natural consequence of this state of things and various approaches have been discussed in the literature [2,5,10,12,14,15,18,19]. Our experimental results have shown [10] that when supported by unlabelled samples much less labelled data is generally required to build a classifier without compromising the classification performance. If only a very limited amount of labelled data is available the results based on random selection of labelled samples show high variability and the performance of the final classifier is more dependent on how reliable the labelled data samples are rather than use of additional unlabelled data. This points to a very interesting discussion point related to the issue of the trade-off between the information content in the observed data (in this case available labels) versus the impact that can be achieved by employing sophisticated data processing algorithms which we will also revisit when discussing approaches dealing with missing feature values.