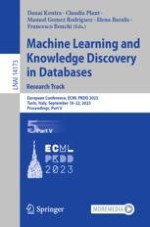
2023 | OriginalPaper | Chapter
MMA: Multi-Metric-Autoencoder for Analyzing High-Dimensional and Incomplete Data
Authors : Cheng Liang, Di Wu, Yi He, Teng Huang, Zhong Chen, Xin Luo
Published in: Machine Learning and Knowledge Discovery in Databases: Research Track
Publisher: Springer Nature Switzerland
Activate our intelligent search to find suitable subject content or patents.
Select sections of text to find matching patents with Artificial Intelligence. powered by
Select sections of text to find additional relevant content using AI-assisted search. powered by