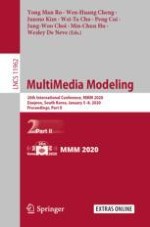
2020 | OriginalPaper | Chapter
Multi-scale Comparison Network for Few-Shot Learning
Authors : Pengfei Chen, Minglei Yuan, Tong Lu
Published in: MultiMedia Modeling
Publisher: Springer International Publishing
Activate our intelligent search to find suitable subject content or patents.
Select sections of text to find matching patents with Artificial Intelligence. powered by
Select sections of text to find additional relevant content using AI-assisted search. powered by