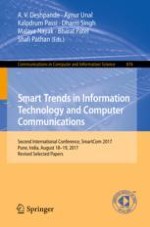
2018 | OriginalPaper | Chapter
Network Intrusion Detection System Using Ensemble of Binary Deep Learning Classifiers
Authors : Aniruddha Parvat, Souradeep Dev, Siddhesh Kadam, Jai Chavan
Published in: Smart Trends in Information Technology and Computer Communications
Publisher: Springer Singapore
Activate our intelligent search to find suitable subject content or patents.
Select sections of text to find matching patents with Artificial Intelligence. powered by
Select sections of text to find additional relevant content using AI-assisted search. powered by