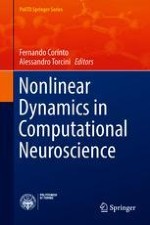
2019 | OriginalPaper | Chapter
Next Generation Neural Mass Models
Authors : Stephen Coombes, Áine Byrne
Published in: Nonlinear Dynamics in Computational Neuroscience
Publisher: Springer International Publishing
Activate our intelligent search to find suitable subject content or patents.
Select sections of text to find matching patents with Artificial Intelligence. powered by
Select sections of text to find additional relevant content using AI-assisted search. powered by