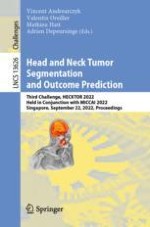
2023 | OriginalPaper | Chapter
Overview of the HECKTOR Challenge at MICCAI 2022: Automatic Head and Neck Tumor Segmentation and Outcome Prediction in PET/CT
Authors : Vincent Andrearczyk, Valentin Oreiller, Moamen Abobakr, Azadeh Akhavanallaf, Panagiotis Balermpas, Sarah Boughdad, Leo Capriotti, Joel Castelli, Catherine Cheze Le Rest, Pierre Decazes, Ricardo Correia, Dina El-Habashy, Hesham Elhalawani, Clifton D. Fuller, Mario Jreige, Yomna Khamis, Agustina La Greca, Abdallah Mohamed, Mohamed Naser, John O. Prior, Su Ruan, Stephanie Tanadini-Lang, Olena Tankyevych, Yazdan Salimi, Martin Vallières, Pierre Vera, Dimitris Visvikis, Kareem Wahid, Habib Zaidi, Mathieu Hatt, Adrien Depeursinge
Published in: Head and Neck Tumor Segmentation and Outcome Prediction
Publisher: Springer Nature Switzerland
Activate our intelligent search to find suitable subject content or patents.
Select sections of text to find matching patents with Artificial Intelligence. powered by
Select sections of text to find additional relevant content using AI-assisted search. powered by