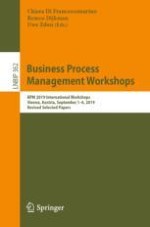
2019 | OriginalPaper | Chapter
Pushing More AI Capabilities into Process Mining to Better Deal with Low-Quality Logs
Authors : Francesco Folino, Luigi Pontieri
Published in: Business Process Management Workshops
Publisher: Springer International Publishing
Activate our intelligent search to find suitable subject content or patents.
Select sections of text to find matching patents with Artificial Intelligence. powered by
Select sections of text to find additional relevant content using AI-assisted search. powered by