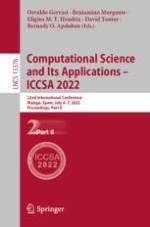
2022 | OriginalPaper | Chapter
Random Forest Based Deep Hybrid Architecture for Histopathological Breast Cancer Images Classification
Authors : Fatima-Zahrae Nakach, Hasnae Zerouaoui, Ali Idri
Published in: Computational Science and Its Applications – ICCSA 2022
Publisher: Springer International Publishing
Activate our intelligent search to find suitable subject content or patents.
Select sections of text to find matching patents with Artificial Intelligence. powered by
Select sections of text to find additional relevant content using AI-assisted search. powered by