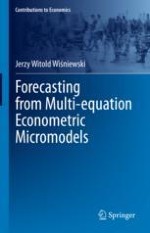
2023 | OriginalPaper | Chapter
1. Single-Equation Econometric Model
Author : Jerzy Witold Wiśniewski
Published in: Forecasting from Multi-equation Econometric Micromodels
Publisher: Springer Nature Switzerland
Activate our intelligent search to find suitable subject content or patents.
Select sections of text to find matching patents with Artificial Intelligence. powered by
Select sections of text to find additional relevant content using AI-assisted search. powered by