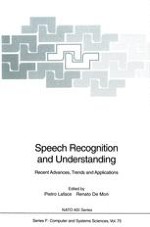
1992 | OriginalPaper | Chapter
Hidden Markov Models for Speech Recognition — Strengths and Limitations
Authors : L. R. Rabiner, B. H. Juang
Published in: Speech Recognition and Understanding
Publisher: Springer Berlin Heidelberg
Included in: Professional Book Archive
Activate our intelligent search to find suitable subject content or patents.
Select sections of text to find matching patents with Artificial Intelligence. powered by
Select sections of text to find additional relevant content using AI-assisted search. powered by
The use of hidden Markov models for speech recognition has become predominant for the last several years, as evidenced by the number of published papers and talks at major speech conferences. The reasons why this method has become so popular are the inherent statistical (mathematically precise) framework, the ease and availability of training algorithms for estimating the parameters of the models from finite training sets of speech data, the flexibility of the resulting recognition system where one can easily change the size, type, or architecture of the models to suit particular words, sounds etc., and the ease of implementation of the overall recognition system. However, although hidden Markov model technology has brought speech recognition system performance to new high levels for a variety of applications, there remain some fundamental areas where aspects of the theory are either inadequate for speech, or for which the assumptions that are made do not apply. Examples of such areas range from the fundamental modeling assumption, i.e. that a maximum likelihood estimate of the model parameters provides the best system performance, to issues involved with inadequate training data which leads to the concepts of parameter tying across states, deleted interpolation and other smoothing methods, etc. Other aspects of the basic hidden Markov modeling methodology which are still not well understood include; ways of integrating new features (e.g. prosodic versus spectral features) into the framework in a consistent and meaningful way; the way to properly model sound durations (both within a state and across states of a model); the way to properly use the information in state transitions; and finally the way in which models can be split or clustered as warranted by the training data. It is the purpose of this paper to examine each of these strengths and limitations and discuss how they affect overall performance of a typical speech recognition system.