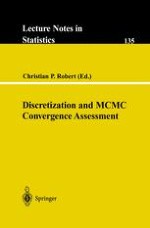
1998 | OriginalPaper | Chapter
Markov Chain Monte Carlo Methods
Authors : Christian P. Robert, Sylvia Richardson
Published in: Discretization and MCMC Convergence Assessment
Publisher: Springer New York
Included in: Professional Book Archive
Activate our intelligent search to find suitable subject content or patents.
Select sections of text to find matching patents with Artificial Intelligence. powered by
Select sections of text to find additional relevant content using AI-assisted search. powered by
As the complexity of the models covered by statistical inference increases, the need for new computational tools gets increasingly pressing. Simulation has always been a natural tool for statisticians (as opposed to numerical analysis) and simulation via Markov chains has been recently exposed as a broad spectrum method, which allows to tackle problems of higher complexity (as shown by the subsequent literature). Although the purpose of this book is to introduce some control techniques for such simulation methods, we feel it is necessary to recall in this chapter the main properties of Markov Chain Monte Carlo (MCMC) algorithms. Moreover, we take the opportunity to introduce notations and our favorite (so-called benchmark) examples, which will be used over and over in the first half of the book. Good introductions to the topic are Gelfand and Smith (1990) seminal paper and Tanner (1996) monograph, as well as Casella and George (1992) and Chib and Greenberg (1996) tutorial papers, and Gelman and Rubin (1992), Geyer (1992) and Besag et al. (1995) surveys, while Neal (1993), Gilks, Richardson and Spiegelhalter (1996), Robert (1996c), Gamerman (1997), Robert and Casella (1998) and Gelfand and Smith (1998) provide deeper entries. In this explosive area of research, many books, monographs and long surveys are currently on their way and it is quite impossible to keep an exact account of the current’ MCMC production!