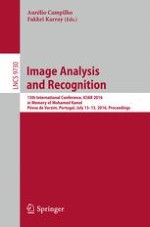
2016 | OriginalPaper | Buchkapitel
Adaptation Approaches in Unsupervised Learning: A Survey of the State-of-the-Art and Future Directions
verfasst von : JunHong Wang, YunQian Miao, Alaa Khamis, Fakhri Karray, Jiye Liang
Erschienen in: Image Analysis and Recognition
Aktivieren Sie unsere intelligente Suche, um passende Fachinhalte oder Patente zu finden.
Wählen Sie Textabschnitte aus um mit Künstlicher Intelligenz passenden Patente zu finden. powered by
Markieren Sie Textabschnitte, um KI-gestützt weitere passende Inhalte zu finden. powered by