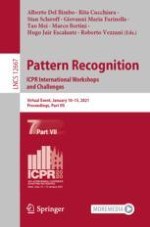
2021 | OriginalPaper | Buchkapitel
Adaptive Future Frame Prediction with Ensemble Network
verfasst von : Wonjik Kim, Masayuki Tanaka, Masatoshi Okutomi, Yoko Sasaki
Erschienen in: Pattern Recognition. ICPR International Workshops and Challenges
Aktivieren Sie unsere intelligente Suche, um passende Fachinhalte oder Patente zu finden.
Wählen Sie Textabschnitte aus um mit Künstlicher Intelligenz passenden Patente zu finden. powered by
Markieren Sie Textabschnitte, um KI-gestützt weitere passende Inhalte zu finden. powered by