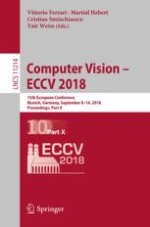
2018 | OriginalPaper | Buchkapitel
Bayesian Semantic Instance Segmentation in Open Set World
verfasst von : Trung Pham, B. G. Vijay Kumar, Thanh-Toan Do, Gustavo Carneiro, Ian Reid
Erschienen in: Computer Vision – ECCV 2018
Aktivieren Sie unsere intelligente Suche, um passende Fachinhalte oder Patente zu finden.
Wählen Sie Textabschnitte aus um mit Künstlicher Intelligenz passenden Patente zu finden. powered by
Markieren Sie Textabschnitte, um KI-gestützt weitere passende Inhalte zu finden. powered by