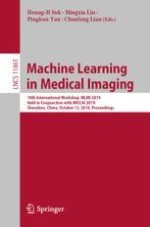
2019 | OriginalPaper | Buchkapitel
Brain MR Image Segmentation in Small Dataset with Adversarial Defense and Task Reorganization
verfasst von : Xuhua Ren, Lichi Zhang, Dongming Wei, Dinggang Shen, Qian Wang
Erschienen in: Machine Learning in Medical Imaging
Aktivieren Sie unsere intelligente Suche, um passende Fachinhalte oder Patente zu finden.
Wählen Sie Textabschnitte aus um mit Künstlicher Intelligenz passenden Patente zu finden. powered by
Markieren Sie Textabschnitte, um KI-gestützt weitere passende Inhalte zu finden. powered by