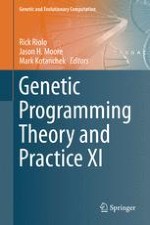
2014 | OriginalPaper | Buchkapitel
1. Extreme Accuracy in Symbolic Regression
verfasst von : Michael F. Korns
Erschienen in: Genetic Programming Theory and Practice XI
Verlag: Springer New York
Aktivieren Sie unsere intelligente Suche, um passende Fachinhalte oder Patente zu finden.
Wählen Sie Textabschnitte aus um mit Künstlicher Intelligenz passenden Patente zu finden. powered by
Markieren Sie Textabschnitte, um KI-gestützt weitere passende Inhalte zu finden. powered by