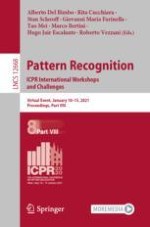
2021 | OriginalPaper | Buchkapitel
Fine-Tuning for One-Look Regression Vehicle Counting in Low-Shot Aerial Datasets
verfasst von : Aneesh Rangnekar, Yi Yao, Matthew Hoffman, Ajay Divakaran
Erschienen in: Pattern Recognition. ICPR International Workshops and Challenges
Aktivieren Sie unsere intelligente Suche, um passende Fachinhalte oder Patente zu finden.
Wählen Sie Textabschnitte aus um mit Künstlicher Intelligenz passenden Patente zu finden. powered by
Markieren Sie Textabschnitte, um KI-gestützt weitere passende Inhalte zu finden. powered by