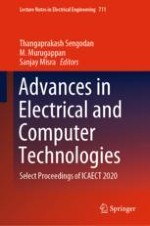
2021 | OriginalPaper | Buchkapitel
Fraudulent Detection in Healthcare Insurance
verfasst von : C. Arunkumar, Srijha Kalyan, Hamsini Ravishankar
Erschienen in: Advances in Electrical and Computer Technologies
Verlag: Springer Nature Singapore
Aktivieren Sie unsere intelligente Suche, um passende Fachinhalte oder Patente zu finden.
Wählen Sie Textabschnitte aus um mit Künstlicher Intelligenz passenden Patente zu finden. powered by
Markieren Sie Textabschnitte, um KI-gestützt weitere passende Inhalte zu finden. powered by