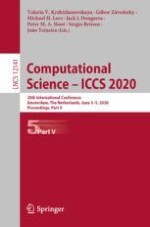
2020 | OriginalPaper | Buchkapitel
Information Theory-Based Feature Selection: Minimum Distribution Similarity with Removed Redundancy
verfasst von : Yu Zhang, Zhuoyi Lin, Chee Keong Kwoh
Erschienen in: Computational Science – ICCS 2020
Aktivieren Sie unsere intelligente Suche, um passende Fachinhalte oder Patente zu finden.
Wählen Sie Textabschnitte aus um mit Künstlicher Intelligenz passenden Patente zu finden. powered by
Markieren Sie Textabschnitte, um KI-gestützt weitere passende Inhalte zu finden. powered by