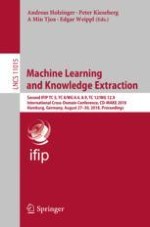
2018 | OriginalPaper | Buchkapitel
Current Advances, Trends and Challenges of Machine Learning and Knowledge Extraction: From Machine Learning to Explainable AI
verfasst von : Andreas Holzinger, Peter Kieseberg, Edgar Weippl, A Min Tjoa
Erschienen in: Machine Learning and Knowledge Extraction
Aktivieren Sie unsere intelligente Suche, um passende Fachinhalte oder Patente zu finden.
Wählen Sie Textabschnitte aus um mit Künstlicher Intelligenz passenden Patente zu finden. powered by
Markieren Sie Textabschnitte, um KI-gestützt weitere passende Inhalte zu finden. powered by