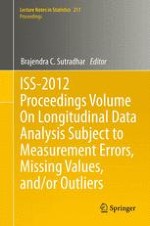
2013 | OriginalPaper | Buchkapitel
Measurement Error Analysis from Independent to Longitudinal Setup
verfasst von : Brajendra C. Sutradhar
Erschienen in: ISS-2012 Proceedings Volume On Longitudinal Data Analysis Subject to Measurement Errors, Missing Values, and/or Outliers
Verlag: Springer New York
Aktivieren Sie unsere intelligente Suche, um passende Fachinhalte oder Patente zu finden.
Wählen Sie Textabschnitte aus um mit Künstlicher Intelligenz passenden Patente zu finden. powered by
Markieren Sie Textabschnitte, um KI-gestützt weitere passende Inhalte zu finden. powered by