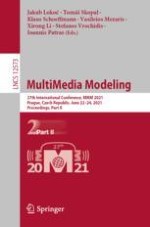
2021 | OriginalPaper | Buchkapitel
MSCANet: Adaptive Multi-scale Context Aggregation Network for Congested Crowd Counting
verfasst von : Yani Zhang, Huailin Zhao, Fangbo Zhou, Qing Zhang, Yanjiao Shi, Lanjun Liang
Erschienen in: MultiMedia Modeling
Aktivieren Sie unsere intelligente Suche, um passende Fachinhalte oder Patente zu finden.
Wählen Sie Textabschnitte aus um mit Künstlicher Intelligenz passenden Patente zu finden. powered by
Markieren Sie Textabschnitte, um KI-gestützt weitere passende Inhalte zu finden. powered by