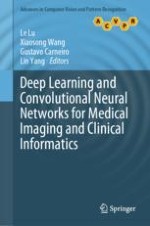
2019 | OriginalPaper | Buchkapitel
1. Pancreas Segmentation in CT and MRI via Task-Specific Network Design and Recurrent Neural Contextual Learning
verfasst von : Jinzheng Cai, Le Lu, Fuyong Xing, Lin Yang
Erschienen in: Deep Learning and Convolutional Neural Networks for Medical Imaging and Clinical Informatics
Aktivieren Sie unsere intelligente Suche, um passende Fachinhalte oder Patente zu finden.
Wählen Sie Textabschnitte aus um mit Künstlicher Intelligenz passenden Patente zu finden. powered by
Markieren Sie Textabschnitte, um KI-gestützt weitere passende Inhalte zu finden. powered by