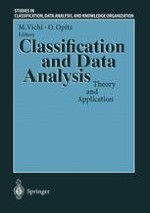
1999 | OriginalPaper | Buchkapitel
Reducing Dimensionality Effects on Kernel Density Estimation: The Bivariate Gaussian Case
verfasst von : Marco Di Marzio, Giovanni Lafratta
Erschienen in: Classification and Data Analysis
Verlag: Springer Berlin Heidelberg
Enthalten in: Professional Book Archive
Aktivieren Sie unsere intelligente Suche, um passende Fachinhalte oder Patente zu finden.
Wählen Sie Textabschnitte aus um mit Künstlicher Intelligenz passenden Patente zu finden. powered by
Markieren Sie Textabschnitte, um KI-gestützt weitere passende Inhalte zu finden. powered by
It is well known that the kernel estimation of multidimensional densities is a difficult task due to the so-called “curse of dimensionality”. The greater the data dimension, the greater is the sample size required to obtain efficient estimates. To reduce such dimensionality effects, we introduce further smoothing sources in addition to the usual bandwidth parametrization. In particular, preliminary kernel estimates are interpreted as smoothed samples and form the basis for successive density estimates, whose average (weights are given by empirical likelihoods of the observed sample) define the proposed sequential density estimator.