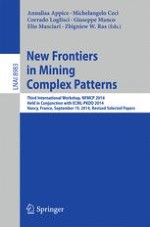
2015 | OriginalPaper | Buchkapitel
Semi-supervised Learning for Multi-target Regression
verfasst von : Jurica Levatić, Michelangelo Ceci, Dragi Kocev, Sašo Džeroski
Erschienen in: New Frontiers in Mining Complex Patterns
Aktivieren Sie unsere intelligente Suche, um passende Fachinhalte oder Patente zu finden.
Wählen Sie Textabschnitte aus um mit Künstlicher Intelligenz passenden Patente zu finden. powered by
Markieren Sie Textabschnitte, um KI-gestützt weitere passende Inhalte zu finden. powered by