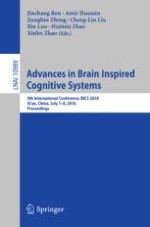
2018 | OriginalPaper | Buchkapitel
Style Neutralization Generative Adversarial Classifier
verfasst von : Haochuan Jiang, Kaizhu Huang, Rui Zhang, Amir Hussain
Erschienen in: Advances in Brain Inspired Cognitive Systems
Aktivieren Sie unsere intelligente Suche, um passende Fachinhalte oder Patente zu finden.
Wählen Sie Textabschnitte aus um mit Künstlicher Intelligenz passenden Patente zu finden. powered by
Markieren Sie Textabschnitte, um KI-gestützt weitere passende Inhalte zu finden. powered by