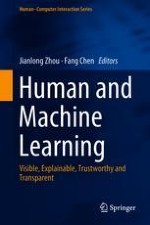
2018 | OriginalPaper | Buchkapitel
1. 2D Transparency Space—Bring Domain Users and Machine Learning Experts Together
verfasst von : Jianlong Zhou, Fang Chen
Erschienen in: Human and Machine Learning
Aktivieren Sie unsere intelligente Suche, um passende Fachinhalte oder Patente zu finden.
Wählen Sie Textabschnitte aus um mit Künstlicher Intelligenz passenden Patente zu finden. powered by
Markieren Sie Textabschnitte, um KI-gestützt weitere passende Inhalte zu finden. powered by