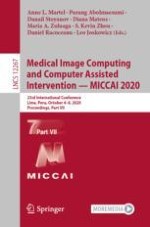
2020 | OriginalPaper | Buchkapitel
A New Metric for Characterizing Dynamic Redundancy of Dense Brain Chronnectome and Its Application to Early Detection of Alzheimer’s Disease
verfasst von : Maryam Ghanbari, Li-Ming Hsu, Zhen Zhou, Amir Ghanbari, Zhanhao Mo, Pew-Thian Yap, Han Zhang, Dinggang Shen
Erschienen in: Medical Image Computing and Computer Assisted Intervention – MICCAI 2020
Aktivieren Sie unsere intelligente Suche, um passende Fachinhalte oder Patente zu finden.
Wählen Sie Textabschnitte aus um mit Künstlicher Intelligenz passenden Patente zu finden. powered by
Markieren Sie Textabschnitte, um KI-gestützt weitere passende Inhalte zu finden. powered by