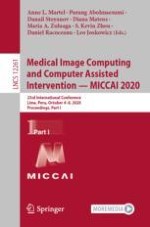
2020 | OriginalPaper | Buchkapitel
Attention, Suggestion and Annotation: A Deep Active Learning Framework for Biomedical Image Segmentation
verfasst von : Haohan Li, Zhaozheng Yin
Erschienen in: Medical Image Computing and Computer Assisted Intervention – MICCAI 2020
Aktivieren Sie unsere intelligente Suche, um passende Fachinhalte oder Patente zu finden.
Wählen Sie Textabschnitte aus um mit Künstlicher Intelligenz passenden Patente zu finden. powered by
Markieren Sie Textabschnitte, um KI-gestützt weitere passende Inhalte zu finden. powered by