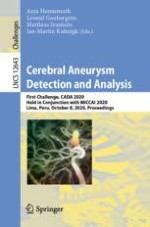
2021 | OriginalPaper | Buchkapitel
Cerebral Aneurysm Detection and Analysis Challenge 2020 (CADA)
verfasst von : Matthias Ivantsits, Leonid Goubergrits, Jan-Martin Kuhnigk, Markus Huellebrand, Jan Brüning, Tabea Kossen, Boris Pfahringer, Jens Schaller, Andreas Spuler, Titus Kuehne, Anja Hennemuth
Erschienen in: Cerebral Aneurysm Detection and Analysis
Aktivieren Sie unsere intelligente Suche, um passende Fachinhalte oder Patente zu finden.
Wählen Sie Textabschnitte aus um mit Künstlicher Intelligenz passenden Patente zu finden. powered by
Markieren Sie Textabschnitte, um KI-gestützt weitere passende Inhalte zu finden. powered by