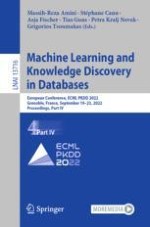
2023 | OriginalPaper | Buchkapitel
Coupling User Preference with External Rewards to Enable Driver-centered and Resource-aware EV Charging Recommendation
verfasst von : Chengyin Li, Zheng Dong, Nathan Fisher, Dongxiao Zhu
Erschienen in: Machine Learning and Knowledge Discovery in Databases
Verlag: Springer Nature Switzerland
Aktivieren Sie unsere intelligente Suche, um passende Fachinhalte oder Patente zu finden.
Wählen Sie Textabschnitte aus um mit Künstlicher Intelligenz passenden Patente zu finden. powered by
Markieren Sie Textabschnitte, um KI-gestützt weitere passende Inhalte zu finden. powered by