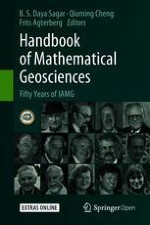
Open Access 2018 | OriginalPaper | Buchkapitel
34. Data Science for Geoscience: Leveraging Mathematical Geosciences with Semantics and Open Data
verfasst von : Xiaogang Ma
Erschienen in: Handbook of Mathematical Geosciences
Aktivieren Sie unsere intelligente Suche, um passende Fachinhalte oder Patente zu finden.
Wählen Sie Textabschnitte aus um mit Künstlicher Intelligenz passenden Patente zu finden. powered by
Markieren Sie Textabschnitte, um KI-gestützt weitere passende Inhalte zu finden. powered by